[5]:
# Templates to setup project enviroment
%run ../template.ipynb
import numpy as np
import os
import pandas as pd
import scenario_tool_nodes.standard_tests as stn
sti = ScenarioToolInterface(api_url="https://stable-api.dance4water.org/api",
results_url="https://stable-sql.dance4water.org/resultsdb/")
sti.login("", "")
import seaborn as sns
# Below are some smaller helper functions
# Scenarios are executed asynchronous
def wait_till_scenario_done(scenario_id):
while True:
status = sti.check_status(scenario_id)
if status["status"] > 6:
print(datetime.datetime.now(), "Scenario complete")
break
if status["status"] < 1:
print(datetime.datetime.now(), "Scenario failed")
sti.show_log(scenario_id)
break
clear_output(wait = True)
print(datetime.datetime.now(), status)
time.sleep(5)
The autoreload extension is already loaded. To reload it, use:
%reload_ext autoreload
Test for Urban Water Cycle Model¶
The urban water cycle catchment¶
The water cycle is assessed within a sub catchment. Sub catchments are subdevided into parcels. A catchment is a geographical unit and may not overlap.
Lot scale¶
Parcel¶
Lot’s in the UWC consume and produce water. A lot in the UWC is a generic unit that can represent a residential lot with a building, a commercial or industrial lot, public spaces including roads, parks or sport ovals.
Following parameters describe a lot in the UWC:
persons (number of people)
area (total are in m2)
roof area (in m2)
impervious area (in m2) (without the roof area)
garden area (in m2) (this area is assumed to be irrigated)
soil profile (see soil profile table)
demand profile (see demand table)
pervious area is calculated as area - roof area - impervious area - graden area
Not all parameters need to be set. Eg. for irrigated public space the number of persons can be 0.
The lot scale considers following internal streams
potable demand > Potable demand is defined by the demand profile and multiplied with the persons on lot
non potable demand
Non-potable demand is defined by the demand profile and multiplied with the persons on lot
black water > Black water is defined by the demand profile and multiplied with the persons on lot
grey water >Grey water is defined by the demand profile and multiplied with the persons on lot
Note: To ensure the mass balance potable + non potable demand = back water + grey water
Areas not covered by lits¶
Areas that are not covered by a parcel as part of a catchment will be lumped into a virtual parcel. Those areas usually includs transport networks. The parameters are caluclated as follows.
impervious area >catchment area * ( average roof cover + average concrete cover + average road cover) - \(\sum\) parcel roof area + \(\sum\) parcel impervious area
pervious area >catchment area - \(\sum\) parcel area - catchment area * (average water cover) - impervious area
Note that if not explicitly defined as part of a parcel green spaces and trees are considered non irrigated.
Streams¶
The following streams are calculated based on a runoff model. The run off model is based on the standard model used in Australia see MUSIC’s rainfall runoff model. T he values are calculated per \(m^2\) and multiplied with the corresponding area’s of the lost.
roof runoff >roof runoff times roof area
impervious runoff >impervious runoff times impervious area
pervious runoff >pervious runoff based times pervious area
evapotranspiration >the evapotranspiration is the sum of the evapotranspiration from roofs, impervious areas, pervious areas and irrigated pervious areas.
infiltration >the infiltration into the deep groundwater layer is based on the soil chracteristics from the pervious area. Infiltration into the deep groundwater layer is not evapotranspirated. The infitration into the pervious soil storage is internally assessed and drives the evapotranspiration and infiltration into the deep groundwater layer.
outdoor demand > the outdoor demand is caculated as the actual evapotranspiration of the pervious area multiplied with a crop factor see
rainfall > rainfall multiplied by the total area of the lot
Runoff model results¶
The results below show the input parameters (default values for Melbourne) for the catchment model and annual flows for 2001 in m or (m3/m2).
[2]:
# Create new project in Melbourne
project_id = sti.create_project()
region_id = sti.get_region( "melbourne")
with open(r"../../resources/boundaries/test_small.geojson", 'r') as file:
geojson_file = json.loads(file.read())
geojson_id = sti.upload_geojson(geojson_file, project_id)
# Set project parameters
sti.update_project(project_id, {
"name": "Water Balance Model v2 Tests",
"active": True,
'region_id': region_id,
"case_study_area_id": geojson_id,
})
[3]:
sti.set_project_data_model(project_id, {"data_model_id": 1, "parameters":
{"micro_climate_grid.grid_size": "20",
"district.source": 1,
"district.layer_name":
"sa1_2011_aust",
"district.epsg_from": "4283",
"parcel.source": 2,
"parcel.layer_name": "property_vic",
"parcel.epsg_from": "4283",
"building.source": 2,
"building.layer_name": "building_geoscape",
"building.epsg_from": "3857",
"landcover_geoscape.raster_file": 3
}})
[4]:
# Add assessment models
lst_model = sti.get_assessment_model("Land Surface Temperature")
water_cycle_model = sti.get_assessment_model("Water Cycle Model v2")
[5]:
# Set assessment models
sti.set_project_assessment_models(project_id, [{"assessment_model_id": lst_model, "parameters" : ""},
{"assessment_model_id": water_cycle_model, "parameters" : ""}])
[6]:
project_id
[6]:
34438
[7]:
baseline_id = sti.create_scenario(project_id, None)
sti.execute_scenario(baseline_id)
[8]:
wait_till_scenario_done(baseline_id)
2020-05-25 21:53:28.607825 {'status': 6, 'status_text': 'PA_RUNNING'}
2020-05-25 21:53:33.782758 Scenario complete
[6]:
# Get results
def build_query_string(table, definitions):
query = 'ogc_fid'
for key, t in definitions[table].items():
query+=','
if t == 'DOUBLEVECTOR':
query+=f'dm_vector_to_string({key}) as {key}'
continue
query+= key
return query
def get_results(scenario_id, results_tables):
while True:
r = sti.run_query(scenario_id, "SELECT view_name, attribute_name, data_type from dynamind_table_definitions")
if r['status'] == 'loaded':
break
if r['status'] == 'error':
print(r)
break
definitions = {}
for entry in r['data']:
if entry['view_name'] not in definitions:
definitions[entry['view_name']] = {}
if entry['attribute_name'] != 'DEFINITION':
definitions[entry['view_name']][entry['attribute_name']] = entry['data_type']
results = {}
for table in results_tables:
while True:
r = sti.run_query(scenario_id, f"SELECT {build_query_string(table, definitions)} from {table}")
if r['status'] == 'loaded':
results[table] = []
for row in r['data']:
converted_row = {}
for key, val in row.items():
converted_row[key] = val
if key in definitions[table]:
if definitions[table][key] == 'DOUBLEVECTOR':
converted_row[key] = np.array([float(d) for d in val.split(" ")])
results[table].append(converted_row)
break
return results
[ ]:
[10]:
# Model paramaters and results
runoff_model_results = pd.DataFrame(get_results(baseline_id, ['wb_demand_profile'])['wb_demand_profile']).T
rr = runoff_model_results.T
runoff_model_results
[10]:
0 | |
---|---|
black_water | 19.0 |
crop_factor | 1.0 |
grey_water | 77.0 |
non_potable_demand_per_person | 19.0 |
ogc_fid | 1.0 |
potable_demand_per_person | 77.0 |
[10]:
# Model paramaters and results
runoff_model_results = pd.DataFrame(get_results(baseline_id, ['wb_soil'])['wb_soil']).T
rr = runoff_model_results.T
runoff_model_results
[10]:
0 | |
---|---|
actual_infiltration | [0.0, 0.0, 0.0, 0.0, 0.0, 0.0, 0.0, 0.0, 0.0, ... |
daily_deep_seepage_rate | 0 |
daily_drainage_rate | 0.05 |
daily_recharge_rate | 0.25 |
effective_evapotranspiration | [0.0, 0.0, 0.0, 0.0, 0.0, 0.0, 0.0, 0.0, 0.0, ... |
evapotranspiration | [0.00277419, 0.00277419, 0.00277419, 0.0027741... |
field_capacity | 0.02 |
groundwater_infiltration | [0.0, 0.0, 0.0, 0.0, 0.0, 0.0, 0.0, 0.0, 0.0, ... |
impervious_evapotranspiration | [0.0004, 0.0, 0.0, 0.0034, 0.0014, 0.0, 0.0, 0... |
impervious_runoff | [0.0, 0.0, 0.0, 0.0, 0.0, 0.0, 0.0, 0.0, 0.0, ... |
impervious_threshold | 0.001 |
infiltration_capacity | 0.2 |
infiltration_exponent | 1 |
initial_groundwater_store | 0.01 |
initial_soil_storage | 0.3 |
ogc_fid | 1 |
outdoor_demand | [0.00237419, 0.00277419, 0.00277419, 0.0, 0.00... |
pervious_evapotranspiration | [0.0004, 0.0, 0.0, 0.0034, 0.0014, 0.0, 0.0, 0... |
pervious_evapotranspiration_irrigated | [0.00277419, 0.00277419, 0.00277419, 0.0034, 0... |
pervious_runoff | [0.0, 0.0, 0.0, 0.0, 0.0, 0.0, 0.0, 0.0, 0.0, ... |
pervious_storage | [0.0, 0.0, 0.0, 0.0, 0.0, 0.0, 0.0, 0.0, 0.0, ... |
possible_infiltration | [2.16, 2.16, 2.16, 0.024, 2.16, 2.16, 2.16, 2.... |
rainfall | [0.0004, 0.0, 0.0, 0.0034, 0.0014, 0.0, 0.0, 0... |
roof_evapotranspiration | [0.0004, 0.0, 0.0, 0.0034, 0.0014, 0.0, 0.0, 0... |
roof_runoff | [0.0, 0.0, 0.0, 0.0, 0.0, 0.0, 0.0, 0.0, 0.0, ... |
soil_store_capacity | 0.03 |
transpiration_capacity | 7 |
Mass balance Impervious surfaces¶
[14]:
def plot_timeseries(vec):
fig, ax = plt.subplots(len(vec),1,figsize=(20, len(vec)*5));
for idx, p in enumerate(vec):
ax[idx].plot(list(range(len(rr[p][0]))), rr[p][0]);
ax[idx].set_title(p);
[15]:
# Mass balance impervious surfaces
print(f"+ {sum(rr['rainfall'][0]):.5f} rainfall")
print(f"- {sum(rr['impervious_runoff'][0]):.5f} impervious_runoff ")
print(f"- {sum(rr['impervious_evapotranspiration'][0]):.5f} impervious_evapotranspiration")
print(f"= {sum((rr['rainfall'][0] - rr['impervious_runoff'][0] -rr['impervious_evapotranspiration'][0])):.5f}")
+ 0.62960 rainfall
- 0.46088 impervious_runoff
- 0.16872 impervious_evapotranspiration
= -0.00000
[17]:
plot_timeseries(['rainfall', 'impervious_runoff', 'impervious_evapotranspiration'])
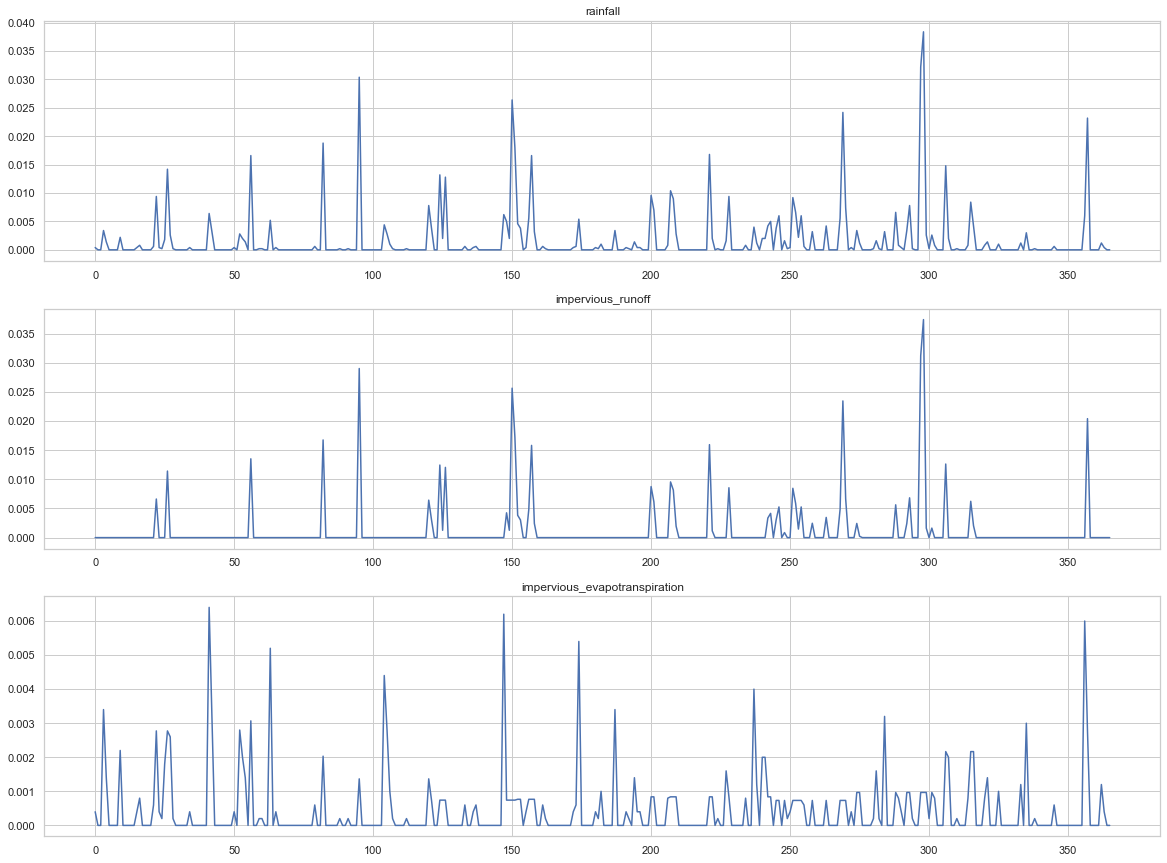
[18]:
# Mass balance roof surfaces
#print(f"rainfall {rr['rainfall'][0]:.3f} - impervious_runoff {rr['impervious_runoff'][0]:.3f} - impervious_evapotranspiration {rr['impervious_evapotranspiration'][0]:.3f} = {(rr['rainfall'][0] - rr['impervious_runoff'][0] -rr['impervious_evapotranspiration'][0]):.3f}")
# currently the initial loss for roof and other impervious surfaces is the same. This might change in a later release
# @todo
roof_evapo = rr['rainfall'][0] - rr['roof_runoff'][0]
print(f"+ {sum(rr['rainfall'][0]):.3f} rainfall")
print(f"- {sum(rr['roof_runoff'][0]):.3f} roof_runoff ")
print(f"- {sum(rr['roof_evapotranspiration'][0]):.3f} roof_evapotranspiration ")
print(f"= {sum(rr['rainfall'][0] - rr['roof_runoff'][0] - rr['roof_evapotranspiration'][0]):.3f}")
+ 0.630 rainfall
- 0.474 roof_runoff
- 0.156 roof_evapotranspiration
= -0.000
[19]:
plot_timeseries(['rainfall', 'roof_runoff', 'roof_evapotranspiration'])
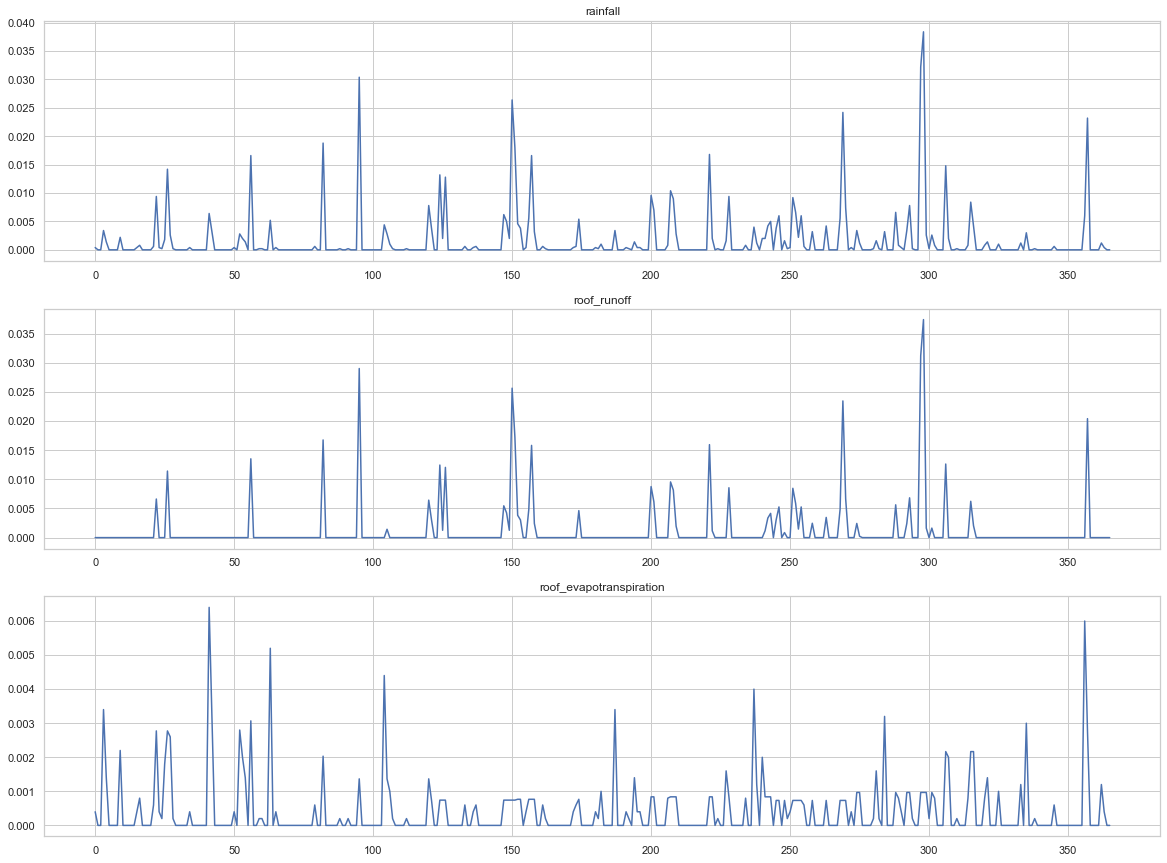
Mass balance Pervious surfaces¶
[20]:
# Mass balance pervious surfaces
print(f"+ {sum(rr['rainfall'][0]):.5f} rainfall")
print(f"- {sum(rr['pervious_runoff'][0]):.5f} pervious_runoff ")
print(f"- {sum(rr['pervious_evapotranspiration'][0]):.5f} pervious_evapotranspiration")
print(f"- {sum(rr['groundwater_infiltration'][0]):.5f} groundwater_infiltration ")
print(f"= {sum(rr['rainfall'][0] - rr['pervious_runoff'][0] -rr['pervious_evapotranspiration'][0]-rr['groundwater_infiltration'][0]):.5f}")
+ 0.62960 rainfall
- 0.02736 pervious_runoff
- 0.32352 pervious_evapotranspiration
- 0.27872 groundwater_infiltration
= -0.00000
[21]:
# rr['rainfall'][0] \
# - rr['pervious_runoff'][0] \
# - rr['actual_infiltration'][0] + rr['effective_evapotranspiration'][0]
[22]:
plot_timeseries(['rainfall', 'pervious_runoff', 'pervious_evapotranspiration', 'groundwater_infiltration'])
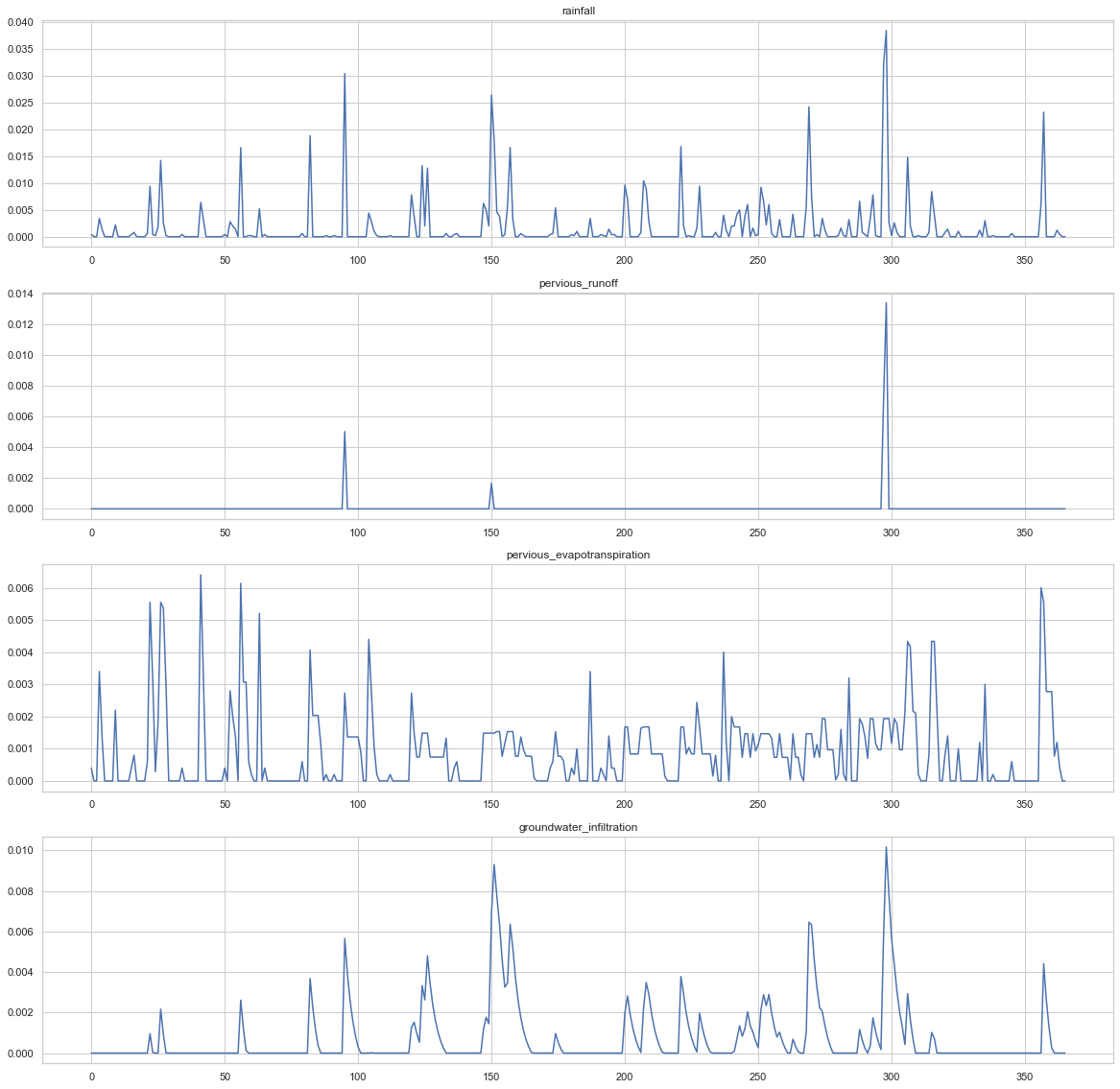
[23]:
# Mass balance pervious irrigated
print(f"+ {sum(rr['rainfall'][0]):.5f} rainfall")
print(f"+ {sum(rr['outdoor_demand'][0]):.5f} outdoor_demand")
print(f"- {sum(rr['pervious_runoff'][0]):.5f} pervious_runoff ")
print(f"- {sum(rr['pervious_evapotranspiration_irrigated'][0]):.5f} pervious_evapotranspiration_irrigated")
print(f"- {sum(rr['groundwater_infiltration'][0]):.5f} groundwater_infiltration ")
print(f"= {sum((rr['rainfall'][0] - rr['pervious_runoff'][0] -rr['pervious_evapotranspiration_irrigated'][0]-rr['groundwater_infiltration'][0]) + rr['outdoor_demand'][0]):.5f}")
+ 0.62960 rainfall
+ 0.44930 outdoor_demand
- 0.02736 pervious_runoff
- 0.77282 pervious_evapotranspiration_irrigated
- 0.27872 groundwater_infiltration
= 0.00000
[24]:
[25]:
plot_timeseries(['rainfall', 'outdoor_demand', 'pervious_runoff', 'pervious_evapotranspiration_irrigated', 'groundwater_infiltration'])
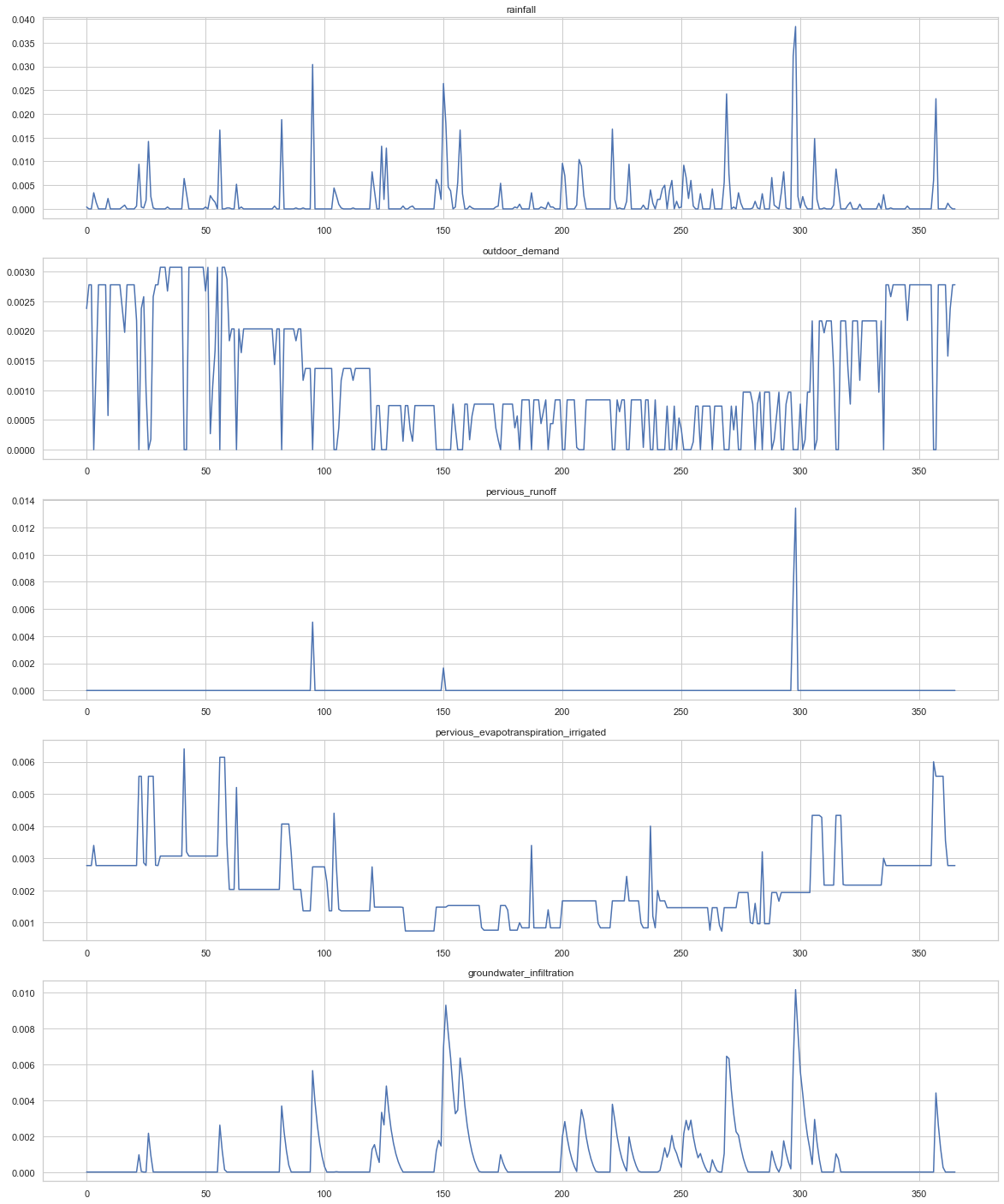
Water Cycle Balance¶
The water balance is assessed on a small urban catchment in Melbourne
[26]:
# Setup new residental development
def create_residental_development():
return {
"node_type_id": sti.get_node_id("Residential"),
"area": geojson_id,
"parameters":
{}
}
def create_storage(demand_stream, inflow_stream,demand_stream_1=0, demand_stream_2=0):
return {
"node_type_id": sti.get_node_id("Lot Scale Storage"),
"area": geojson_id,
"parameters":
{
"dance4water_template_id.equation" : 1,
"dance4water_inflow_stream.equation": inflow_stream,
"dance4water_demand_stream.equation": demand_stream,
"dance4water_demand_stream_1.equation": demand_stream_1,
"dance4water_demand_stream_2.equation": demand_stream_2,
"dance4water_volume.equation": 5
}
}
def wb_catchment(name, geojson_id):
return [{
"node_type_id": sti.get_node_id("Copy Feature"),
"area": geojson_id,
"parameters":
{'dance4water_copy_feature.from_view': 'dance4water',
'dance4water_copy_feature.to_view': 'sub_catchment',
'dance4water_copy_feature.add_link': '0',
'dance4water_copy_feature.copy_features': '1'}
},
{"node_type_id": sti.get_node_id("SQL query"),
"area": geojson_id,
"parameters":
{'dance4water_sql_query.attribute': 'sub_catchment.name',
'dance4water_sql_query.query': f'UPDATE sub_catchment set name = \'{name}\' WHERE name is null',
'dance4water_sql_query.attribute_type': 'DOUBLE'}
}]
def setup_and_start_project(name,
with_RWHT_storage = False,
with_grey_and_rwht_storage=False,
with_residual_storage=False):
scenario_id = sti.create_scenario(project_id, baseline_id, name)
nodes = []
# nodes+=wb_catchment("default", geojson_id)
nodes.append(create_residental_development())
if with_RWHT_storage:
nodes.append(create_storage(int(LotStream.outdoor_demand), int(LotStream.roof_runoff)))
if with_residual_storage:
nodes.append(create_storage(int(LotStream.outdoor_demand),
int(LotStream.roof_runoff),
int(LotStream.non_potable_demand)))
if with_grey_and_rwht_storage:
nodes.append(create_storage(int(LotStream.outdoor_demand), int(LotStream.roof_runoff)))
nodes.append(create_storage(int(LotStream.outdoor_demand), int(LotStream.grey_water)))
sti.set_scenario_workflow(scenario_id, nodes)
sti.execute_scenario(scenario_id)
return scenario_id
[29]:
scenarios = {}
scenarios["Residential"] = setup_and_start_project("Residential")
[30]:
wait_till_scenario_done(scenarios["Residential"])
2020-05-25 21:54:39.100631 {'status': 6, 'status_text': 'PA_RUNNING'}
2020-05-25 21:54:44.293305 Scenario complete
Mass balance Impervious surfaces¶
[31]:
# Mass balance all units are in SI
def calcuate_mass_balance(df: pd.DataFrame):
df["mass_balance"]= df["rainfall"] \
+ df["potable_demand"] \
+ df["non_potable_demand"] \
+ df["outdoor_demand"] \
- df["grey_water"] \
- df["black_water"] \
- df["evapotranspiration"] \
- df["impervious_runoff"] \
- df["pervious_runoff"] \
- df["roof_runoff"] \
- df["infiltration"]
df["mass_balance_runoff_only"] = df["rainfall"] \
+ df["outdoor_demand"] \
- df["evapotranspiration"] \
- df["impervious_runoff"] \
- df["pervious_runoff"] \
- df["roof_runoff"] \
- df["infiltration"]
return df
def inflow(df: pd.DataFrame):
df["inflow"]= df["rainfall"] \
+ df["potable_demand"] \
+ df["non_potable_demand"] \
+ df["outdoor_demand"]
def outflow(df: pd.DataFrame):
df["outflow"] = df["evapotranspiration"] \
+ df["grey_water"] \
+ df["black_water"] \
+ df["impervious_runoff"] \
+ df["pervious_runoff"] \
+ df["roof_runoff"] \
+ df["infiltration"]
from IPython.display import display, HTML
def print_parcel_mass_balance(df):
display(HTML(df.filter(["area",
"persons",
"impervious_area",
"roof_area" ,
"garden_area",
"outdoor_imp",
"potable_demand",
"outdoor_demand",
"non_potable_demand",
"grey_water",
"black_water",
"rainfall",
"evapotranspiration",
"impervious_runoff",
"pervious_runoff",
"roof_runoff",
"infiltration",
"mass_balance_runoff_only",
"mass_balance",
"wb_lot_template_id"
]).to_html()))
[33]:
# Download results
scenario_results = get_results(scenarios["Residential"], ['parcel', 'wb_soil'])
lot_residential = pd.DataFrame(scenario_results['parcel'])
lot_residential = calcuate_mass_balance(lot_residential)
print_parcel_mass_balance(lot_residential)
area | persons | impervious_area | roof_area | garden_area | outdoor_imp | potable_demand | outdoor_demand | non_potable_demand | grey_water | black_water | rainfall | evapotranspiration | impervious_runoff | pervious_runoff | roof_runoff | infiltration | mass_balance_runoff_only | mass_balance | wb_lot_template_id | |
---|---|---|---|---|---|---|---|---|---|---|---|---|---|---|---|---|---|---|---|---|
0 | 628.974360 | 2.0 | None | 377.384616 | 150.953846 | 100.635898 | 56.364 | 67.823171 | 13.908 | 56.364 | 13.908 | 396.002257 | 192.525340 | 46.380798 | 4.129480 | 178.715501 | 42.074310 | -1.563194e-13 | -1.563194e-13 | 1 |
1 | 639.210270 | 4.0 | None | 383.526162 | 153.410465 | 102.273643 | 112.728 | 68.926924 | 27.816 | 112.728 | 27.816 | 402.446786 | 195.658491 | 47.135598 | 4.196683 | 181.623912 | 42.759026 | 5.684342e-13 | 5.684342e-13 | 1 |
2 | 644.454460 | 3.0 | None | 386.672676 | 154.669070 | 103.112714 | 84.546 | 69.492412 | 20.862 | 84.546 | 20.862 | 405.748528 | 197.263707 | 47.522306 | 4.231113 | 183.113985 | 43.109828 | -2.131628e-13 | -9.947598e-14 | 1 |
3 | 644.201990 | 3.0 | None | 386.521194 | 154.608478 | 103.072318 | 84.546 | 69.465188 | 20.862 | 84.546 | 20.862 | 405.589573 | 197.186427 | 47.503689 | 4.229456 | 183.042249 | 43.092939 | -1.207923e-13 | -6.394885e-14 | 1 |
4 | 644.454460 | 1.0 | None | 386.672676 | 154.669070 | 103.112714 | 28.182 | 69.492412 | 6.954 | 28.182 | 6.954 | 405.748528 | 197.263707 | 47.522306 | 4.231113 | 183.113985 | 43.109828 | -4.618528e-13 | -4.050094e-13 | 1 |
5 | 644.033676 | 2.0 | None | 386.420206 | 154.568082 | 103.045388 | 56.364 | 69.447038 | 13.908 | 56.364 | 13.908 | 405.483603 | 197.134908 | 47.491277 | 4.228351 | 182.994425 | 43.081680 | -2.415845e-13 | -1.278977e-13 | 1 |
6 | 644.454460 | 1.0 | None | 386.672676 | 154.669070 | 103.112714 | 28.182 | 69.492412 | 6.954 | 28.182 | 6.954 | 405.748528 | 197.263707 | 47.522306 | 4.231113 | 183.113985 | 43.109828 | 2.842171e-13 | 2.842171e-13 | 1 |
7 | 643.865363 | 4.0 | None | 386.319218 | 154.527687 | 103.018458 | 112.728 | 69.428889 | 27.816 | 112.728 | 27.816 | 405.377633 | 197.083388 | 47.478866 | 4.227246 | 182.946601 | 43.070421 | 6.394885e-14 | 6.394885e-14 | 1 |
8 | 644.454460 | 3.0 | None | 386.672676 | 154.669070 | 103.112714 | 84.546 | 69.492412 | 20.862 | 84.546 | 20.862 | 405.748528 | 197.263707 | 47.522306 | 4.231113 | 183.113985 | 43.109828 | 5.684342e-14 | 1.705303e-13 | 1 |
9 | 643.697050 | 1.0 | None | 386.218230 | 154.487292 | 102.991528 | 28.182 | 69.410739 | 6.954 | 28.182 | 6.954 | 405.271662 | 197.031868 | 47.466454 | 4.226141 | 182.898777 | 43.059162 | -7.815970e-14 | -7.815970e-14 | 1 |
10 | 644.454460 | 4.0 | None | 386.672676 | 154.669070 | 103.112714 | 112.728 | 69.492412 | 27.816 | 112.728 | 27.816 | 405.748528 | 197.263707 | 47.522306 | 4.231113 | 183.113985 | 43.109828 | 1.421085e-13 | 1.421085e-13 | 1 |
11 | 643.528736 | 3.0 | None | 386.117242 | 154.446897 | 102.964598 | 84.546 | 69.392590 | 20.862 | 84.546 | 20.862 | 405.165692 | 196.980348 | 47.454043 | 4.225036 | 182.850952 | 43.047903 | -1.207923e-13 | -1.207923e-13 | 1 |
12 | 644.454460 | 3.0 | None | 386.672676 | 154.669070 | 103.112714 | 84.546 | 69.492412 | 20.862 | 84.546 | 20.862 | 405.748528 | 197.263707 | 47.522306 | 4.231113 | 183.113985 | 43.109828 | 5.684342e-13 | 6.821210e-13 | 1 |
13 | 643.360423 | 2.0 | None | 386.016254 | 154.406502 | 102.937668 | 56.364 | 69.374440 | 13.908 | 56.364 | 13.908 | 405.059722 | 196.928829 | 47.441631 | 4.223931 | 182.803128 | 43.036644 | -2.913225e-13 | -2.913225e-13 | 1 |
14 | 644.454460 | 1.0 | None | 386.672676 | 154.669070 | 103.112714 | 28.182 | 69.492412 | 6.954 | 28.182 | 6.954 | 405.748528 | 197.263707 | 47.522306 | 4.231113 | 183.113985 | 43.109828 | 3.552714e-14 | 3.552714e-14 | 1 |
15 | 643.192110 | 2.0 | None | 385.915266 | 154.366106 | 102.910738 | 56.364 | 69.356291 | 13.908 | 56.364 | 13.908 | 404.953752 | 196.877309 | 47.429220 | 4.222825 | 182.755304 | 43.025385 | 6.394885e-14 | 6.394885e-14 | 1 |
16 | 644.454460 | 3.0 | None | 386.672676 | 154.669070 | 103.112714 | 84.546 | 69.492412 | 20.862 | 84.546 | 20.862 | 405.748528 | 197.263707 | 47.522306 | 4.231113 | 183.113985 | 43.109828 | 3.339551e-13 | 2.771117e-13 | 1 |
17 | 643.023796 | 3.0 | None | 385.814278 | 154.325711 | 102.883807 | 84.546 | 69.338141 | 20.862 | 84.546 | 20.862 | 404.847782 | 196.825789 | 47.416808 | 4.221720 | 182.707480 | 43.014126 | 6.252776e-13 | 6.252776e-13 | 1 |
18 | 644.454460 | 1.0 | None | 386.672676 | 154.669070 | 103.112714 | 28.182 | 69.492412 | 6.954 | 28.182 | 6.954 | 405.748528 | 197.263707 | 47.522306 | 4.231113 | 183.113985 | 43.109828 | -5.115908e-13 | -5.115908e-13 | 1 |
19 | 642.855483 | 4.0 | None | 385.713290 | 154.285316 | 102.856877 | 112.728 | 69.319992 | 27.816 | 112.728 | 27.816 | 404.741812 | 196.774270 | 47.404397 | 4.220615 | 182.659655 | 43.002867 | -1.705303e-13 | -1.705303e-13 | 1 |
20 | 644.454460 | 2.0 | None | 386.672676 | 154.669070 | 103.112714 | 56.364 | 69.492412 | 13.908 | 56.364 | 13.908 | 405.748528 | 197.263707 | 47.522306 | 4.231113 | 183.113985 | 43.109828 | -3.126388e-13 | -2.557954e-13 | 1 |
21 | 642.687170 | 4.0 | None | 385.612302 | 154.244921 | 102.829947 | 112.728 | 69.301843 | 27.816 | 112.728 | 27.816 | 404.635842 | 196.722750 | 47.391985 | 4.219510 | 182.611831 | 42.991608 | 1.634248e-13 | 1.634248e-13 | 1 |
22 | 644.454460 | 2.0 | None | 386.672676 | 154.669070 | 103.112714 | 56.364 | 69.492412 | 13.908 | 56.364 | 13.908 | 405.748528 | 197.263707 | 47.522306 | 4.231113 | 183.113985 | 43.109828 | -8.526513e-14 | -2.842171e-14 | 1 |
23 | 642.518856 | 1.0 | None | 385.511314 | 154.204526 | 102.803017 | 28.182 | 69.283693 | 6.954 | 28.182 | 6.954 | 404.529872 | 196.671230 | 47.379574 | 4.218405 | 182.564007 | 42.980349 | -3.197442e-13 | -3.197442e-13 | 1 |
24 | 644.454460 | 2.0 | None | 386.672676 | 154.669070 | 103.112714 | 56.364 | 69.492412 | 13.908 | 56.364 | 13.908 | 405.748528 | 197.263707 | 47.522306 | 4.231113 | 183.113985 | 43.109828 | 3.197442e-13 | 3.197442e-13 | 1 |
25 | 642.350543 | 4.0 | None | 385.410326 | 154.164130 | 102.776087 | 112.728 | 69.265544 | 27.816 | 112.728 | 27.816 | 404.423902 | 196.619710 | 47.367162 | 4.217300 | 182.516183 | 42.969090 | 9.947598e-14 | 9.947598e-14 | 1 |
26 | 644.454460 | 1.0 | None | 386.672676 | 154.669070 | 103.112714 | 28.182 | 69.492412 | 6.954 | 28.182 | 6.954 | 405.748528 | 197.263707 | 47.522306 | 4.231113 | 183.113985 | 43.109828 | 5.684342e-14 | 0.000000e+00 | 1 |
27 | 642.182230 | 1.0 | None | 385.309338 | 154.123735 | 102.749157 | 28.182 | 69.247394 | 6.954 | 28.182 | 6.954 | 404.317932 | 196.568191 | 47.354751 | 4.216195 | 182.468359 | 42.957831 | -6.536993e-13 | -6.536993e-13 | 1 |
28 | 629.627695 | 4.0 | None | 377.776617 | 151.110647 | 100.740431 | 112.728 | 67.893621 | 27.816 | 112.728 | 27.816 | 396.413597 | 192.725322 | 46.428975 | 4.133769 | 178.901139 | 42.118013 | 3.694822e-13 | 3.694822e-13 | 1 |
29 | 597.765026 | 1.0 | None | 358.659016 | 143.463606 | 95.642404 | 28.182 | 64.457826 | 6.954 | 28.182 | 6.954 | 376.352860 | 182.972347 | 44.079410 | 3.924578 | 169.847744 | 39.986607 | -8.526513e-14 | -2.842171e-14 | 1 |
30 | 4920.163308 | NaN | None | NaN | NaN | 2043.436272 | 0.000 | 0.000000 | 0.000 | 0.000 | 0.000 | 3097.734818 | 1275.455995 | 941.773332 | 78.695489 | 0.000000 | 801.810002 | -1.818989e-12 | -1.818989e-12 | 1 |
[34]:
# Download results
scenario_results = get_results(baseline_id, ['parcel', 'wb_soil'])
lot_residential = pd.DataFrame(scenario_results['parcel'])
lot_residential = calcuate_mass_balance(lot_residential)
print_parcel_mass_balance(lot_residential)
area | persons | impervious_area | roof_area | garden_area | outdoor_imp | potable_demand | outdoor_demand | non_potable_demand | grey_water | black_water | rainfall | evapotranspiration | impervious_runoff | pervious_runoff | roof_runoff | infiltration | mass_balance_runoff_only | mass_balance | wb_lot_template_id | |
---|---|---|---|---|---|---|---|---|---|---|---|---|---|---|---|---|---|---|---|---|
0 | 442.291056 | None | None | 167.515769 | None | None | 0.0 | 0.0 | 0.0 | 0.0 | 0.0 | 278.466449 | 115.034208 | 0.0 | 7.516728 | 79.329319 | 76.586193 | 9.947598e-14 | 9.947598e-14 | 1 |
1 | 224.502687 | None | None | NaN | None | None | 0.0 | 0.0 | 0.0 | 0.0 | 0.0 | 141.346891 | 72.631353 | 0.0 | 6.141476 | 0.000000 | 62.574063 | 7.105427e-14 | 7.105427e-14 | 1 |
2 | 441.455388 | None | None | 159.516779 | None | None | 0.0 | 0.0 | 0.0 | 0.0 | 0.0 | 277.940312 | 116.103558 | 0.0 | 7.712687 | 75.541291 | 78.582776 | 4.263256e-14 | 4.263256e-14 | 1 |
3 | 472.476300 | None | None | 207.092681 | None | None | 0.0 | 0.0 | 0.0 | 0.0 | 0.0 | 297.471079 | 118.171257 | 0.0 | 7.259811 | 98.071492 | 73.968520 | -9.947598e-14 | -9.947598e-14 | 1 |
4 | 229.969140 | None | None | 134.562405 | None | None | 0.0 | 0.0 | 0.0 | 0.0 | 0.0 | 144.788570 | 51.862765 | 0.0 | 2.609938 | 63.723815 | 26.592052 | -3.552714e-14 | -3.552714e-14 | 1 |
5 | 452.063127 | None | None | 230.621051 | None | None | 0.0 | 0.0 | 0.0 | 0.0 | 0.0 | 284.618945 | 107.626530 | 0.0 | 6.057750 | 109.213664 | 61.721001 | -1.705303e-13 | -1.705303e-13 | 1 |
6 | 457.705755 | None | None | 187.208012 | None | None | 0.0 | 0.0 | 0.0 | 0.0 | 0.0 | 288.171543 | 116.723045 | 0.0 | 7.399712 | 88.654843 | 75.393944 | 5.684342e-14 | 5.684342e-14 | 1 |
7 | 482.809249 | None | None | 166.472338 | None | None | 0.0 | 0.0 | 0.0 | 0.0 | 0.0 | 303.976703 | 128.317456 | 0.0 | 8.653684 | 78.835189 | 88.170374 | 1.421085e-14 | 1.421085e-14 | 1 |
8 | 441.958057 | None | None | 189.436842 | None | None | 0.0 | 0.0 | 0.0 | 0.0 | 0.0 | 278.256793 | 111.255039 | 0.0 | 6.907948 | 89.710334 | 70.383472 | 2.984279e-13 | 2.984279e-13 | 1 |
9 | 445.505812 | None | None | 144.138375 | None | None | 0.0 | 0.0 | 0.0 | 0.0 | 0.0 | 280.490459 | 119.989597 | 0.0 | 8.244181 | 68.258643 | 83.998037 | 8.526513e-14 | 8.526513e-14 | 1 |
10 | 382.776842 | None | None | 145.744326 | None | None | 0.0 | 0.0 | 0.0 | 0.0 | 0.0 | 240.996300 | 99.426481 | 0.0 | 6.484240 | 69.019163 | 66.066415 | -7.105427e-14 | -7.105427e-14 | 1 |
11 | 452.442852 | None | None | 361.484372 | None | None | 0.0 | 0.0 | 0.0 | 0.0 | 0.0 | 284.858019 | 85.831817 | 0.0 | 2.488252 | 171.185730 | 25.352221 | 1.207923e-13 | 1.207923e-13 | 1 |
12 | 222.966199 | None | None | 278.984428 | None | None | 0.0 | 0.0 | 0.0 | 0.0 | 0.0 | 140.379519 | 25.408733 | 0.0 | -1.532430 | 132.116784 | -15.613569 | 1.172396e-13 | 1.172396e-13 | 1 |
13 | 445.498908 | None | None | 188.906277 | None | None | 0.0 | 0.0 | 0.0 | 0.0 | 0.0 | 280.486113 | 112.489440 | 0.0 | 7.019325 | 89.459078 | 71.518269 | 8.526513e-14 | 8.526513e-14 | 1 |
14 | 225.551023 | None | None | 212.172490 | None | None | 0.0 | 0.0 | 0.0 | 0.0 | 0.0 | 142.006924 | 37.434934 | 0.0 | 0.365982 | 100.477103 | 3.728905 | 5.417888e-14 | 5.417888e-14 | 1 |
15 | 437.377090 | None | None | 208.049934 | None | None | 0.0 | 0.0 | 0.0 | 0.0 | 0.0 | 275.372616 | 106.655597 | 0.0 | 6.273453 | 98.524812 | 63.918754 | 6.394885e-14 | 6.394885e-14 | 1 |
16 | 724.515066 | None | None | 325.786031 | None | None | 0.0 | 0.0 | 0.0 | 0.0 | 0.0 | 456.154685 | 179.831829 | 0.0 | 10.907596 | 154.280305 | 111.134955 | 1.421085e-13 | 1.421085e-13 | 1 |
17 | 497.251203 | None | None | 182.697084 | None | None | 0.0 | 0.0 | 0.0 | 0.0 | 0.0 | 313.069358 | 130.272341 | 0.0 | 8.604914 | 86.518633 | 87.673469 | -2.557954e-13 | -2.557954e-13 | 1 |
18 | 450.056993 | None | None | 346.204658 | None | None | 0.0 | 0.0 | 0.0 | 0.0 | 0.0 | 283.355883 | 87.619055 | 0.0 | 2.840975 | 163.949818 | 28.946035 | 3.552714e-15 | 3.552714e-15 | 1 |
19 | 437.247884 | None | None | 207.009283 | None | None | 0.0 | 0.0 | 0.0 | 0.0 | 0.0 | 275.291268 | 106.788089 | 0.0 | 6.298387 | 98.031998 | 64.172794 | 1.421085e-13 | 1.421085e-13 | 1 |
20 | 478.855767 | None | None | 169.127638 | None | None | 0.0 | 0.0 | 0.0 | 0.0 | 0.0 | 301.487591 | 126.593700 | 0.0 | 8.472895 | 80.092641 | 86.328355 | -2.273737e-13 | -2.273737e-13 | 1 |
21 | 435.432567 | None | None | 268.633170 | None | None | 0.0 | 0.0 | 0.0 | 0.0 | 0.0 | 274.148344 | 95.879757 | 0.0 | 4.562949 | 127.214808 | 46.490829 | 1.207923e-13 | 1.207923e-13 | 1 |
22 | 445.803138 | None | None | 203.006181 | None | None | 0.0 | 0.0 | 0.0 | 0.0 | 0.0 | 280.677655 | 110.226351 | 0.0 | 6.641932 | 96.136276 | 67.673097 | -1.563194e-13 | -1.563194e-13 | 1 |
23 | 454.924256 | None | None | 205.008282 | None | None | 0.0 | 0.0 | 0.0 | 0.0 | 0.0 | 286.420312 | 112.841904 | 0.0 | 6.836679 | 97.084397 | 69.657331 | 5.684342e-14 | 5.684342e-14 | 1 |
24 | 464.169786 | None | None | 140.536237 | None | None | 0.0 | 0.0 | 0.0 | 0.0 | 0.0 | 292.241297 | 126.631089 | 0.0 | 8.853291 | 66.552803 | 90.204115 | 1.421085e-13 | 1.421085e-13 | 1 |
25 | 477.695923 | None | None | 304.590795 | None | None | 0.0 | 0.0 | 0.0 | 0.0 | 0.0 | 300.757353 | 103.530503 | 0.0 | 4.735448 | 144.243020 | 48.248381 | 9.947598e-14 | 9.947598e-14 | 1 |
26 | 464.519505 | None | None | 184.597204 | None | None | 0.0 | 0.0 | 0.0 | 0.0 | 0.0 | 292.461481 | 119.364707 | 0.0 | 7.657530 | 87.418460 | 78.020785 | 2.557954e-13 | 2.557954e-13 | 1 |
27 | 491.121048 | None | None | 351.120162 | None | None | 0.0 | 0.0 | 0.0 | 0.0 | 0.0 | 309.209812 | 100.080873 | 0.0 | 3.829852 | 166.277620 | 39.021468 | 7.815970e-14 | 7.815970e-14 | 1 |
28 | 460.921047 | None | None | 240.476642 | None | None | 0.0 | 0.0 | 0.0 | 0.0 | 0.0 | 290.195891 | 108.841596 | 0.0 | 6.030458 | 113.880910 | 61.442927 | 0.000000e+00 | 0.000000e+00 | 1 |
29 | 340.486856 | None | None | 221.780167 | None | None | 0.0 | 0.0 | 0.0 | 0.0 | 0.0 | 214.370525 | 73.009964 | 0.0 | 3.247330 | 105.026946 | 33.086285 | -7.105427e-15 | -7.105427e-15 | 1 |
30 | 446.773795 | None | None | 242.479600 | None | None | 0.0 | 0.0 | 0.0 | 0.0 | 0.0 | 281.288781 | 103.929198 | 0.0 | 5.588654 | 114.829438 | 56.941492 | -3.907985e-13 | -3.907985e-13 | 1 |
31 | 444.564346 | None | None | 136.611036 | None | None | 0.0 | 0.0 | 0.0 | 0.0 | 0.0 | 279.897712 | 120.945725 | 0.0 | 8.424343 | 64.693972 | 85.833672 | 2.842171e-14 | 2.842171e-14 | 1 |
32 | 454.703067 | None | None | 181.610980 | None | None | 0.0 | 0.0 | 0.0 | 0.0 | 0.0 | 286.281051 | 116.689027 | 0.0 | 7.470683 | 86.004294 | 76.117047 | -8.526513e-14 | -8.526513e-14 | 1 |
33 | 719.259607 | None | None | 238.721034 | None | None | 0.0 | 0.0 | 0.0 | 0.0 | 0.0 | 452.845849 | 192.713605 | 0.0 | 13.145570 | 113.049519 | 133.937155 | 4.263256e-13 | 4.263256e-13 | 1 |
34 | 37.077187 | None | None | NaN | None | None | 0.0 | 0.0 | 0.0 | 0.0 | 0.0 | 23.343797 | 11.995252 | 0.0 | 1.014280 | 0.000000 | 10.334265 | 0.000000e+00 | 0.000000e+00 | 1 |
35 | 483.390176 | None | None | 213.210084 | None | None | 0.0 | 0.0 | 0.0 | 0.0 | 0.0 | 304.342455 | 120.677556 | 0.0 | 7.391023 | 100.968469 | 75.305407 | 2.273737e-13 | 2.273737e-13 | 1 |
36 | 444.032897 | None | None | 289.821623 | None | None | 0.0 | 0.0 | 0.0 | 0.0 | 0.0 | 279.563112 | 95.113410 | 0.0 | 4.218590 | 137.248882 | 42.982229 | 1.207923e-13 | 1.207923e-13 | 1 |
37 | 479.787211 | None | None | 169.512469 | None | None | 0.0 | 0.0 | 0.0 | 0.0 | 0.0 | 302.074028 | 126.830589 | 0.0 | 8.487848 | 80.274883 | 86.480708 | 2.700062e-13 | 2.700062e-13 | 1 |
38 | 7742.298968 | None | None | NaN | None | None | 0.0 | 0.0 | 0.0 | 0.0 | 0.0 | 4874.551430 | 2504.796960 | 0.0 | 211.797641 | 0.000000 | 2157.956830 | -2.728484e-12 | -2.728484e-12 | 1 |
[35]:
# Display scenario
# import IPython
# from IPython.display import IFrame
# url = f'https://staging.wsc-scenario.org.au/project/{project_id}'
# IFrame(src=url, width=1000, height=800)
Storages¶
Storages at lot scale can be connect to any of the internal streams listed above as inflow or demand. (demand stream \(\neq\) inflow stream). The storage overflow will become the new reduced inflow stream and the provided water stream will become the new reduced demand stream. Eg in case of a rainwater harvesting tank the roof runoff will be reduced by the storage as well as the outdoor demand.
Those modified streams can be used as input for a new storage. The first storage can simulate for example a rainwater tank providing non potable water for toilet flushing linked with a grey water tank that if the rainwater tank is empty will provide additional water.
Singe RWHT¶
For this test we use a 5m3 storage to provide outdoor demand from roof runoff
[36]:
scenarios["Residential with RWHT"] = setup_and_start_project("Residential with RWHT", with_RWHT_storage=True)
[37]:
wait_till_scenario_done(scenarios["Residential with RWHT"])
2020-05-25 21:55:39.860797 {'status': 6, 'status_text': 'PA_RUNNING'}
2020-05-25 21:55:45.050787 Scenario complete
[38]:
# Download results
scenario_results = get_results(scenarios["Residential with RWHT"], ['parcel', 'wb_soil', 'wb_lot_storages'])
lot_residential_with_rwht = pd.DataFrame(scenario_results['parcel'])
lot_residential_with_rwht = calcuate_mass_balance(lot_residential_with_rwht)
print_parcel_mass_balance(lot_residential_with_rwht)
area | persons | impervious_area | roof_area | garden_area | outdoor_imp | potable_demand | outdoor_demand | non_potable_demand | grey_water | black_water | rainfall | evapotranspiration | impervious_runoff | pervious_runoff | roof_runoff | infiltration | mass_balance_runoff_only | mass_balance | wb_lot_template_id | |
---|---|---|---|---|---|---|---|---|---|---|---|---|---|---|---|---|---|---|---|---|
0 | 628.974360 | 4.0 | None | 377.384616 | 150.953846 | 100.635898 | 112.728 | 24.868304 | 27.816 | 112.728 | 27.816 | 396.002257 | 192.525340 | 46.380798 | 4.129480 | 133.869310 | 42.074310 | 1.891325e+00 | 1.891325e+00 | 1 |
1 | 639.210270 | 2.0 | None | 383.526162 | 153.410465 | 102.273643 | 56.364 | 25.517120 | 13.908 | 56.364 | 13.908 | 402.446786 | 195.658491 | 47.135598 | 4.196683 | 136.373374 | 42.759026 | 1.840734e+00 | 1.840734e+00 | 1 |
2 | 644.454460 | 4.0 | None | 386.672676 | 154.669070 | 103.112714 | 112.728 | 25.849529 | 27.816 | 112.728 | 27.816 | 405.748528 | 197.263707 | 47.522306 | 4.231113 | 137.656288 | 43.109828 | 1.814815e+00 | 1.814815e+00 | 1 |
3 | 644.201990 | 2.0 | None | 386.521194 | 154.608478 | 103.072318 | 56.364 | 25.833526 | 13.908 | 56.364 | 13.908 | 405.589573 | 197.186427 | 47.503689 | 4.229456 | 137.594525 | 43.092939 | 1.816063e+00 | 1.816063e+00 | 1 |
4 | 644.454460 | 4.0 | None | 386.672676 | 154.669070 | 103.112714 | 112.728 | 25.849529 | 27.816 | 112.728 | 27.816 | 405.748528 | 197.263707 | 47.522306 | 4.231113 | 137.656288 | 43.109828 | 1.814815e+00 | 1.814815e+00 | 1 |
5 | 644.033676 | 3.0 | None | 386.420206 | 154.568082 | 103.045388 | 84.546 | 25.822858 | 20.862 | 84.546 | 20.862 | 405.483603 | 197.134908 | 47.491277 | 4.228351 | 137.553350 | 43.081680 | 1.816895e+00 | 1.816895e+00 | 1 |
6 | 644.454460 | 4.0 | None | 386.672676 | 154.669070 | 103.112714 | 112.728 | 25.849529 | 27.816 | 112.728 | 27.816 | 405.748528 | 197.263707 | 47.522306 | 4.231113 | 137.656288 | 43.109828 | 1.814815e+00 | 1.814815e+00 | 1 |
7 | 643.865363 | 2.0 | None | 386.319218 | 154.527687 | 103.018458 | 56.364 | 25.812189 | 13.908 | 56.364 | 13.908 | 405.377633 | 197.083388 | 47.478866 | 4.227246 | 137.512174 | 43.070421 | 1.817727e+00 | 1.817727e+00 | 1 |
8 | 644.454460 | 4.0 | None | 386.672676 | 154.669070 | 103.112714 | 112.728 | 25.849529 | 27.816 | 112.728 | 27.816 | 405.748528 | 197.263707 | 47.522306 | 4.231113 | 137.656288 | 43.109828 | 1.814815e+00 | 1.814815e+00 | 1 |
9 | 643.697050 | 2.0 | None | 386.218230 | 154.487292 | 102.991528 | 56.364 | 25.801520 | 13.908 | 56.364 | 13.908 | 405.271662 | 197.031868 | 47.466454 | 4.226141 | 137.470999 | 43.059162 | 1.818558e+00 | 1.818558e+00 | 1 |
10 | 644.454460 | 3.0 | None | 386.672676 | 154.669070 | 103.112714 | 84.546 | 25.849529 | 20.862 | 84.546 | 20.862 | 405.748528 | 197.263707 | 47.522306 | 4.231113 | 137.656288 | 43.109828 | 1.814815e+00 | 1.814815e+00 | 1 |
11 | 643.528736 | 4.0 | None | 386.117242 | 154.446897 | 102.964598 | 112.728 | 25.790851 | 27.816 | 112.728 | 27.816 | 405.165692 | 196.980348 | 47.454043 | 4.225036 | 137.429823 | 43.047903 | 1.819390e+00 | 1.819390e+00 | 1 |
12 | 644.454460 | 3.0 | None | 386.672676 | 154.669070 | 103.112714 | 84.546 | 25.849529 | 20.862 | 84.546 | 20.862 | 405.748528 | 197.263707 | 47.522306 | 4.231113 | 137.656288 | 43.109828 | 1.814815e+00 | 1.814815e+00 | 1 |
13 | 643.360423 | 1.0 | None | 386.016254 | 154.406502 | 102.937668 | 28.182 | 25.780183 | 6.954 | 28.182 | 6.954 | 405.059722 | 196.928829 | 47.441631 | 4.223931 | 137.388648 | 43.036644 | 1.820222e+00 | 1.820222e+00 | 1 |
14 | 644.454460 | 1.0 | None | 386.672676 | 154.669070 | 103.112714 | 28.182 | 25.849529 | 6.954 | 28.182 | 6.954 | 405.748528 | 197.263707 | 47.522306 | 4.231113 | 137.656288 | 43.109828 | 1.814815e+00 | 1.814815e+00 | 1 |
15 | 643.192110 | 3.0 | None | 385.915266 | 154.366106 | 102.910738 | 84.546 | 25.769514 | 20.862 | 84.546 | 20.862 | 404.953752 | 196.877309 | 47.429220 | 4.222825 | 137.347473 | 43.025385 | 1.821054e+00 | 1.821054e+00 | 1 |
16 | 644.454460 | 4.0 | None | 386.672676 | 154.669070 | 103.112714 | 112.728 | 25.849529 | 27.816 | 112.728 | 27.816 | 405.748528 | 197.263707 | 47.522306 | 4.231113 | 137.656288 | 43.109828 | 1.814815e+00 | 1.814815e+00 | 1 |
17 | 643.023796 | 2.0 | None | 385.814278 | 154.325711 | 102.883807 | 56.364 | 25.758845 | 13.908 | 56.364 | 13.908 | 404.847782 | 196.825789 | 47.416808 | 4.221720 | 137.306297 | 43.014126 | 1.821886e+00 | 1.821886e+00 | 1 |
18 | 644.454460 | 1.0 | None | 386.672676 | 154.669070 | 103.112714 | 28.182 | 25.849529 | 6.954 | 28.182 | 6.954 | 405.748528 | 197.263707 | 47.522306 | 4.231113 | 137.656288 | 43.109828 | 1.814815e+00 | 1.814815e+00 | 1 |
19 | 642.855483 | 4.0 | None | 385.713290 | 154.285316 | 102.856877 | 112.728 | 25.748176 | 27.816 | 112.728 | 27.816 | 404.741812 | 196.774270 | 47.404397 | 4.220615 | 137.265122 | 43.002867 | 1.822718e+00 | 1.822718e+00 | 1 |
20 | 644.454460 | 1.0 | None | 386.672676 | 154.669070 | 103.112714 | 28.182 | 25.849529 | 6.954 | 28.182 | 6.954 | 405.748528 | 197.263707 | 47.522306 | 4.231113 | 137.656288 | 43.109828 | 1.814815e+00 | 1.814815e+00 | 1 |
21 | 642.687170 | 4.0 | None | 385.612302 | 154.244921 | 102.829947 | 112.728 | 25.737508 | 27.816 | 112.728 | 27.816 | 404.635842 | 196.722750 | 47.391985 | 4.219510 | 137.223947 | 42.991608 | 1.823550e+00 | 1.823550e+00 | 1 |
22 | 644.454460 | 3.0 | None | 386.672676 | 154.669070 | 103.112714 | 84.546 | 25.849529 | 20.862 | 84.546 | 20.862 | 405.748528 | 197.263707 | 47.522306 | 4.231113 | 137.656288 | 43.109828 | 1.814815e+00 | 1.814815e+00 | 1 |
23 | 642.518856 | 3.0 | None | 385.511314 | 154.204526 | 102.803017 | 84.546 | 25.726839 | 20.862 | 84.546 | 20.862 | 404.529872 | 196.671230 | 47.379574 | 4.218405 | 137.182771 | 42.980349 | 1.824382e+00 | 1.824382e+00 | 1 |
24 | 644.454460 | 4.0 | None | 386.672676 | 154.669070 | 103.112714 | 112.728 | 25.849529 | 27.816 | 112.728 | 27.816 | 405.748528 | 197.263707 | 47.522306 | 4.231113 | 137.656288 | 43.109828 | 1.814815e+00 | 1.814815e+00 | 1 |
25 | 642.350543 | 2.0 | None | 385.410326 | 154.164130 | 102.776087 | 56.364 | 25.716170 | 13.908 | 56.364 | 13.908 | 404.423902 | 196.619710 | 47.367162 | 4.217300 | 137.141596 | 42.969090 | 1.825214e+00 | 1.825214e+00 | 1 |
26 | 644.454460 | 1.0 | None | 386.672676 | 154.669070 | 103.112714 | 28.182 | 25.849529 | 6.954 | 28.182 | 6.954 | 405.748528 | 197.263707 | 47.522306 | 4.231113 | 137.656288 | 43.109828 | 1.814815e+00 | 1.814815e+00 | 1 |
27 | 642.182230 | 1.0 | None | 385.309338 | 154.123735 | 102.749157 | 28.182 | 25.705501 | 6.954 | 28.182 | 6.954 | 404.317932 | 196.568191 | 47.354751 | 4.216195 | 137.100420 | 42.957831 | 1.826045e+00 | 1.826045e+00 | 1 |
28 | 629.627695 | 4.0 | None | 377.776617 | 151.110647 | 100.740431 | 112.728 | 24.909717 | 27.816 | 112.728 | 27.816 | 396.413597 | 192.725322 | 46.428975 | 4.133769 | 134.029139 | 42.118013 | 1.888096e+00 | 1.888096e+00 | 1 |
29 | 597.765026 | 4.0 | None | 358.659016 | 143.463606 | 95.642404 | 112.728 | 23.037035 | 27.816 | 112.728 | 27.816 | 376.352860 | 182.972347 | 44.079410 | 3.924578 | 126.381379 | 39.986607 | 2.045575e+00 | 2.045575e+00 | 1 |
30 | 4920.163308 | NaN | None | NaN | NaN | 2043.436272 | 0.000 | 0.000000 | 0.000 | 0.000 | 0.000 | 3097.734818 | 1275.455995 | 941.773332 | 78.695489 | 0.000000 | 801.810002 | -1.818989e-12 | -1.818989e-12 | 1 |
[39]:
def plot_timeseries_df(df, vec):
fig, ax = plt.subplots(len(vec),1,figsize=(20, len(vec)*5));
for idx, p in enumerate(vec):
ax[idx].plot(list(range(len(df[p]))), df[p]);
ax[idx].set_title(p);
Storage behaviour of a single storage¶
Note the error in the mass balance is equal to the remaining water in the RWHT
[40]:
# Plot lot storage 1
lot_storages = pd.DataFrame(scenario_results['wb_lot_storages'])
plot_timeseries_df(lot_storages.loc[0], ['storage_behaviour', 'provided_volume'])
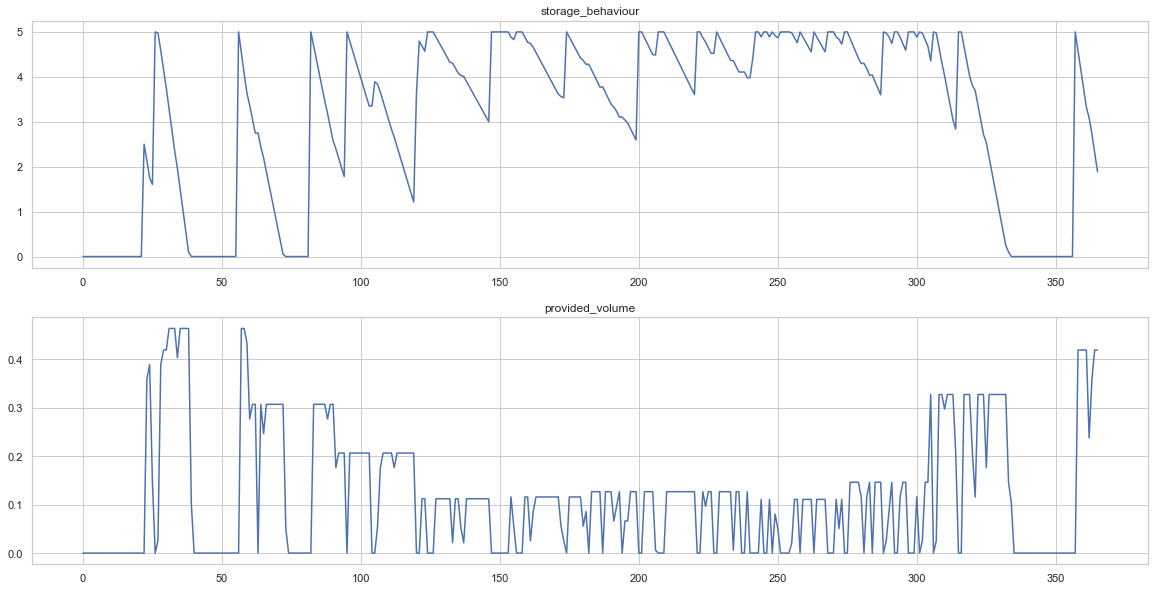
[41]:
# Plot lot storage 1
ls = pd.DataFrame(lot_storages.sum()).T
plot_timeseries_df(ls.loc[0], ['storage_behaviour', 'provided_volume'])
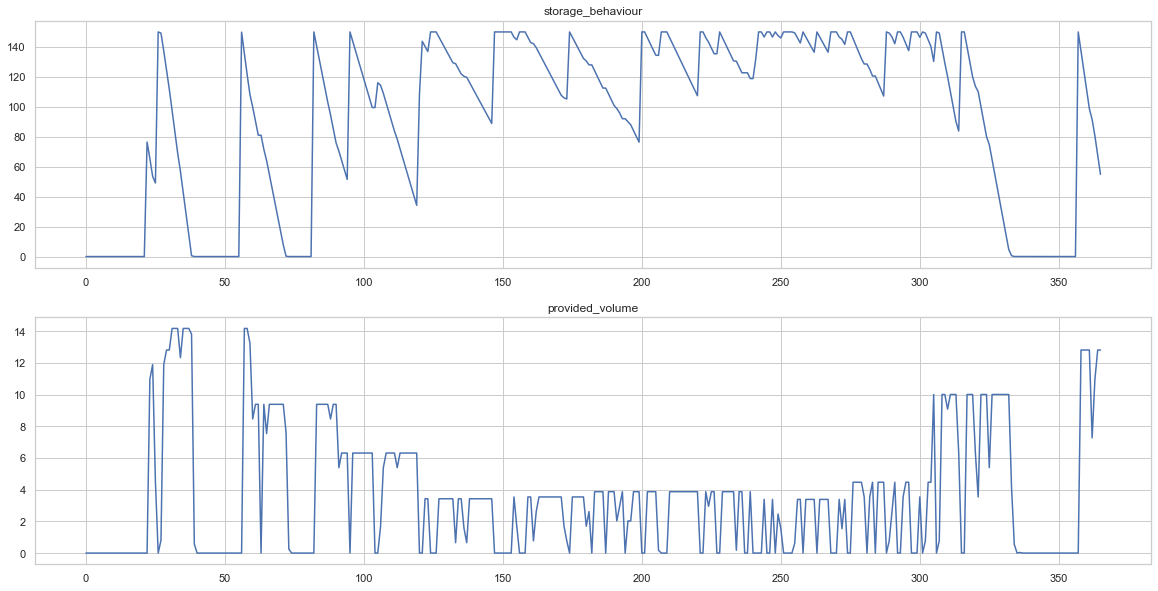
[42]:
# sti.run_query(scenarios["Residential with RWHT"],
# "SELECT CAST(strftime('%s', '2001-01-01 00:00:00') as INTEGER) as t_start, 60*60*24 as t_step, dm_vector_to_string(dm_sum_vectors(storage_behaviour)) as t from wb_lot_storages")
Cascading RWHT¶
For this test we use a 5m3 storage to provide non-potable water from roof runoff and grey water
[43]:
scenarios["Residential with RWHT and Grey Water"] = setup_and_start_project("Residential with RWHT and Grey Water", with_grey_and_rwht_storage=True)
[44]:
wait_till_scenario_done(scenarios["Residential with RWHT and Grey Water"])
2020-05-25 21:56:41.783774 {'status': 6, 'status_text': 'PA_RUNNING'}
2020-05-25 21:56:46.965306 Scenario complete
[45]:
# Download results
scenario_results = get_results(scenarios["Residential with RWHT and Grey Water"], ['parcel', 'wb_soil', 'wb_lot_storages'])
lot_residential_with_grey = pd.DataFrame(scenario_results['parcel'])
lot_residential_with_grey = calcuate_mass_balance(lot_residential_with_grey)
print_parcel_mass_balance(lot_residential_with_grey)
area | persons | impervious_area | roof_area | garden_area | outdoor_imp | potable_demand | outdoor_demand | non_potable_demand | grey_water | black_water | rainfall | evapotranspiration | impervious_runoff | pervious_runoff | roof_runoff | infiltration | mass_balance_runoff_only | mass_balance | wb_lot_template_id | |
---|---|---|---|---|---|---|---|---|---|---|---|---|---|---|---|---|---|---|---|---|
0 | 628.974360 | 3.0 | None | 377.384616 | 150.953846 | 100.635898 | 84.546 | 2.836747 | 20.862 | 58.839183 | 20.862 | 396.002257 | 192.525340 | 46.380798 | 4.129480 | 133.869310 | 42.074310 | -2.014023e+01 | 5.566584e+00 | 1 |
1 | 639.210270 | 3.0 | None | 383.526162 | 153.410465 | 102.273643 | 84.546 | 2.965616 | 20.862 | 58.453574 | 20.862 | 402.446786 | 195.658491 | 47.135598 | 4.196683 | 136.373374 | 42.759026 | -2.071077e+01 | 5.381656e+00 | 1 |
2 | 644.454460 | 1.0 | None | 386.672676 | 154.669070 | 103.112714 | 28.182 | 12.825096 | 6.954 | 14.387566 | 6.954 | 405.748528 | 197.263707 | 47.522306 | 4.231113 | 137.656288 | 43.109828 | -1.120962e+01 | 2.584815e+00 | 1 |
3 | 644.201990 | 3.0 | None | 386.521194 | 154.608478 | 103.072318 | 84.546 | 3.028461 | 20.862 | 58.265525 | 20.862 | 405.589573 | 197.186427 | 47.503689 | 4.229456 | 137.594525 | 43.092939 | -2.098900e+01 | 5.291473e+00 | 1 |
4 | 644.454460 | 1.0 | None | 386.672676 | 154.669070 | 103.112714 | 28.182 | 12.825096 | 6.954 | 14.387566 | 6.954 | 405.748528 | 197.263707 | 47.522306 | 4.231113 | 137.656288 | 43.109828 | -1.120962e+01 | 2.584815e+00 | 1 |
5 | 644.033676 | 4.0 | None | 386.420206 | 154.568082 | 103.045388 | 112.728 | 1.332342 | 27.816 | 83.237485 | 27.816 | 405.483603 | 197.134908 | 47.491277 | 4.228351 | 137.553350 | 43.081680 | -2.267362e+01 | 6.816895e+00 | 1 |
6 | 644.454460 | 1.0 | None | 386.672676 | 154.669070 | 103.112714 | 28.182 | 12.825096 | 6.954 | 14.387566 | 6.954 | 405.748528 | 197.263707 | 47.522306 | 4.231113 | 137.656288 | 43.109828 | -1.120962e+01 | 2.584815e+00 | 1 |
7 | 643.865363 | 4.0 | None | 386.319218 | 154.527687 | 103.018458 | 112.728 | 1.330223 | 27.816 | 83.246035 | 27.816 | 405.377633 | 197.083388 | 47.478866 | 4.227246 | 137.512174 | 43.070421 | -2.266424e+01 | 6.817727e+00 | 1 |
8 | 644.454460 | 1.0 | None | 386.672676 | 154.669070 | 103.112714 | 28.182 | 12.825096 | 6.954 | 14.387566 | 6.954 | 405.748528 | 197.263707 | 47.522306 | 4.231113 | 137.656288 | 43.109828 | -1.120962e+01 | 2.584815e+00 | 1 |
9 | 643.697050 | 3.0 | None | 386.218230 | 154.487292 | 102.991528 | 84.546 | 3.022104 | 20.862 | 58.284547 | 20.862 | 405.271662 | 197.031868 | 47.466454 | 4.226141 | 137.470999 | 43.059162 | -2.096086e+01 | 5.300595e+00 | 1 |
10 | 644.454460 | 2.0 | None | 386.672676 | 154.669070 | 103.112714 | 56.364 | 6.012734 | 13.908 | 34.987204 | 13.908 | 405.748528 | 197.263707 | 47.522306 | 4.231113 | 137.656288 | 43.109828 | -1.802198e+01 | 3.354815e+00 | 1 |
11 | 643.528736 | 4.0 | None | 386.117242 | 154.446897 | 102.964598 | 112.728 | 1.325985 | 27.816 | 83.263134 | 27.816 | 405.165692 | 196.980348 | 47.454043 | 4.225036 | 137.429823 | 43.047903 | -2.264548e+01 | 6.819390e+00 | 1 |
12 | 644.454460 | 2.0 | None | 386.672676 | 154.669070 | 103.112714 | 56.364 | 6.012734 | 13.908 | 34.987204 | 13.908 | 405.748528 | 197.263707 | 47.522306 | 4.231113 | 137.656288 | 43.109828 | -1.802198e+01 | 3.354815e+00 | 1 |
13 | 643.360423 | 4.0 | None | 386.016254 | 154.406502 | 102.937668 | 112.728 | 1.323866 | 27.816 | 83.271684 | 27.816 | 405.059722 | 196.928829 | 47.441631 | 4.223931 | 137.388648 | 43.036644 | -2.263609e+01 | 6.820222e+00 | 1 |
14 | 644.454460 | 4.0 | None | 386.672676 | 154.669070 | 103.112714 | 112.728 | 1.337640 | 27.816 | 83.216111 | 27.816 | 405.748528 | 197.263707 | 47.522306 | 4.231113 | 137.656288 | 43.109828 | -2.269707e+01 | 6.814815e+00 | 1 |
15 | 643.192110 | 1.0 | None | 385.915266 | 154.366106 | 102.910738 | 28.182 | 12.754906 | 6.954 | 14.397393 | 6.954 | 404.953752 | 196.877309 | 47.429220 | 4.222825 | 137.347473 | 43.025385 | -1.119355e+01 | 2.591054e+00 | 1 |
16 | 644.454460 | 3.0 | None | 386.672676 | 154.669070 | 103.112714 | 84.546 | 3.031640 | 20.862 | 58.256014 | 20.862 | 405.748528 | 197.263707 | 47.522306 | 4.231113 | 137.656288 | 43.109828 | -2.100307e+01 | 5.286912e+00 | 1 |
17 | 643.023796 | 1.0 | None | 385.814278 | 154.325711 | 102.883807 | 28.182 | 12.745548 | 6.954 | 14.398703 | 6.954 | 404.847782 | 196.825789 | 47.416808 | 4.221720 | 137.306297 | 43.014126 | -1.119141e+01 | 2.591886e+00 | 1 |
18 | 644.454460 | 1.0 | None | 386.672676 | 154.669070 | 103.112714 | 28.182 | 12.825096 | 6.954 | 14.387566 | 6.954 | 405.748528 | 197.263707 | 47.522306 | 4.231113 | 137.656288 | 43.109828 | -1.120962e+01 | 2.584815e+00 | 1 |
19 | 642.855483 | 1.0 | None | 385.713290 | 154.285316 | 102.856877 | 28.182 | 12.736189 | 6.954 | 14.400013 | 6.954 | 404.741812 | 196.774270 | 47.404397 | 4.220615 | 137.265122 | 43.002867 | -1.118927e+01 | 2.592718e+00 | 1 |
20 | 644.454460 | 3.0 | None | 386.672676 | 154.669070 | 103.112714 | 84.546 | 3.031640 | 20.862 | 58.256014 | 20.862 | 405.748528 | 197.263707 | 47.522306 | 4.231113 | 137.656288 | 43.109828 | -2.100307e+01 | 5.286912e+00 | 1 |
21 | 642.687170 | 3.0 | None | 385.612302 | 154.244921 | 102.829947 | 84.546 | 3.009390 | 20.862 | 58.322592 | 20.862 | 404.635842 | 196.722750 | 47.391985 | 4.219510 | 137.223947 | 42.991608 | -2.090457e+01 | 5.318840e+00 | 1 |
22 | 644.454460 | 4.0 | None | 386.672676 | 154.669070 | 103.112714 | 112.728 | 1.337640 | 27.816 | 83.216111 | 27.816 | 405.748528 | 197.263707 | 47.522306 | 4.231113 | 137.656288 | 43.109828 | -2.269707e+01 | 6.814815e+00 | 1 |
23 | 642.518856 | 4.0 | None | 385.511314 | 154.204526 | 102.803017 | 112.728 | 1.313271 | 27.816 | 83.314432 | 27.816 | 404.529872 | 196.671230 | 47.379574 | 4.218405 | 137.182771 | 42.980349 | -2.258919e+01 | 6.824382e+00 | 1 |
24 | 644.454460 | 4.0 | None | 386.672676 | 154.669070 | 103.112714 | 112.728 | 1.337640 | 27.816 | 83.216111 | 27.816 | 405.748528 | 197.263707 | 47.522306 | 4.231113 | 137.656288 | 43.109828 | -2.269707e+01 | 6.814815e+00 | 1 |
25 | 642.350543 | 3.0 | None | 385.410326 | 154.164130 | 102.776087 | 84.546 | 3.005152 | 20.862 | 58.335273 | 20.862 | 404.423902 | 196.619710 | 47.367162 | 4.217300 | 137.141596 | 42.969090 | -2.088580e+01 | 5.324922e+00 | 1 |
26 | 644.454460 | 4.0 | None | 386.672676 | 154.669070 | 103.112714 | 112.728 | 1.337640 | 27.816 | 83.216111 | 27.816 | 405.748528 | 197.263707 | 47.522306 | 4.231113 | 137.656288 | 43.109828 | -2.269707e+01 | 6.814815e+00 | 1 |
27 | 642.182230 | 4.0 | None | 385.309338 | 154.123735 | 102.749157 | 112.728 | 1.309033 | 27.816 | 83.331531 | 27.816 | 404.317932 | 196.568191 | 47.354751 | 4.216195 | 137.100420 | 42.957831 | -2.257042e+01 | 6.826045e+00 | 1 |
28 | 629.627695 | 3.0 | None | 377.776617 | 151.110647 | 100.740431 | 84.546 | 2.844972 | 20.862 | 58.814570 | 20.862 | 396.413597 | 192.725322 | 46.428975 | 4.133769 | 134.029139 | 42.118013 | -2.017665e+01 | 5.554780e+00 | 1 |
29 | 597.765026 | 4.0 | None | 358.659016 | 143.463606 | 95.642404 | 112.728 | 0.749823 | 27.816 | 85.440788 | 27.816 | 376.352860 | 182.972347 | 44.079410 | 3.924578 | 126.381379 | 39.986607 | -2.024164e+01 | 7.045575e+00 | 1 |
30 | 4920.163308 | NaN | None | NaN | NaN | 2043.436272 | 0.000 | 0.000000 | 0.000 | 0.000000 | 0.000 | 3097.734818 | 1275.455995 | 941.773332 | 78.695489 | 0.000000 | 801.810002 | -1.818989e-12 | -1.818989e-12 | 1 |
Storage behaviour RWHT¶
[46]:
# Plot lot storage 1
lot_storages = pd.DataFrame(scenario_results['wb_lot_storages'])
plot_timeseries_df(lot_storages.loc[0], ['storage_behaviour', 'provided_volume'])
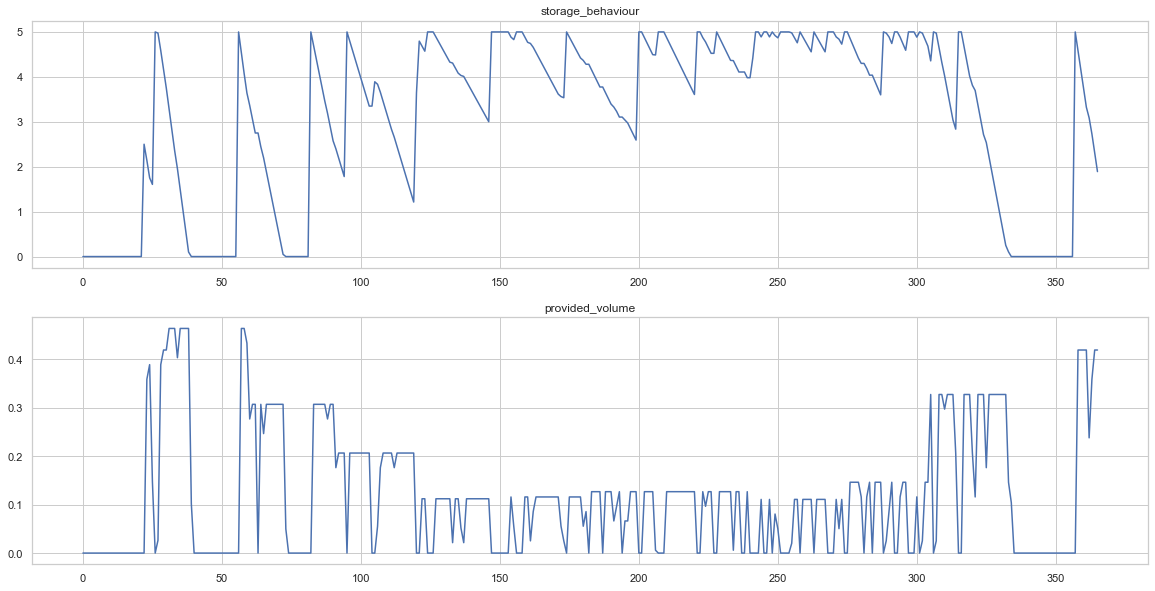
[ ]:
[47]:
# Fit porpuse residual demand
# Multilpe sources
Storage behaviour grey water tank¶
[48]:
# Plot lot storage 2
plot_timeseries_df(lot_storages.loc[1], ['storage_behaviour', 'provided_volume'])
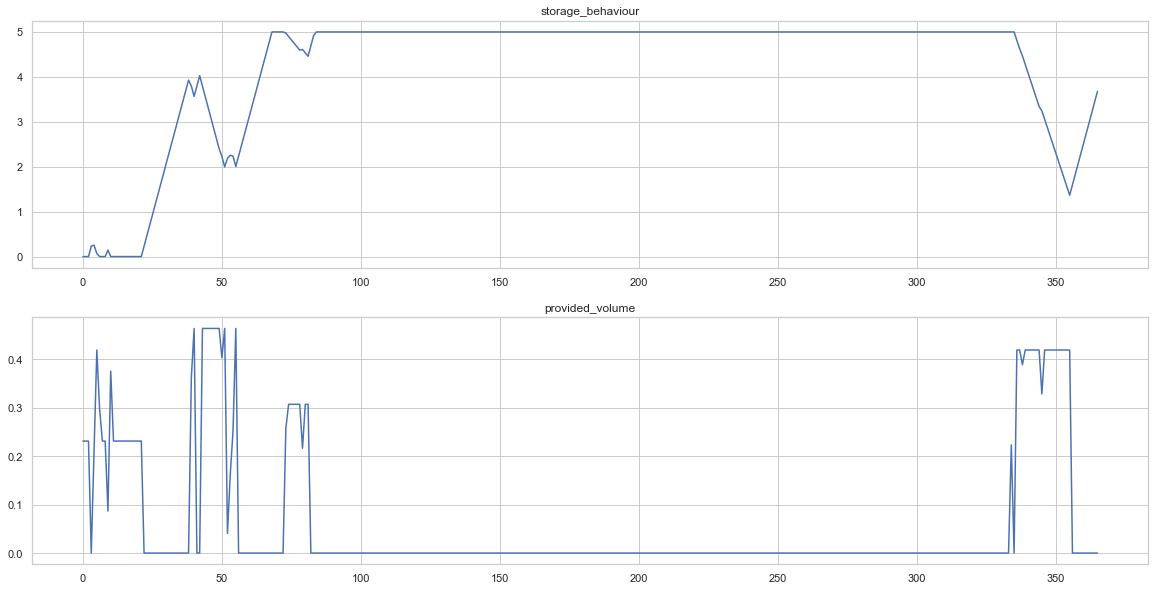
[49]:
sti.run_query(scenarios["Residential with RWHT and Grey Water"],
"SELECT dm_vector_sum(wb_lot_storages.provided_volume) as volume, CASE inflow_stream_id WHEN 1 THEN 'potable demand'WHEN 2 THEN 'non potable demand'WHEN 3 THEN 'outdoor demand'WHEN 4 THEN 'black water'WHEN 5 THEN 'grey water'WHEN 6 THEN 'roof runoff'WHEN 7 THEN 'impervious runoff'WHEN 8 THEN 'pervious runoff' WHEN 9 THEN 'evapotranspiration' WHEN 10 THEN 'infiltration' WHEN 11 THEN 'rainfall' ELSE 'Other' END as inflow from wb_lot_storages LEFT JOIN wb_storages on storage_id = wb_storages.ogc_fid group by storage_id")
[49]:
{'cached': False, 'data': 'Run query', 'status': 'submitted'}
Residual Supply from Storages¶
[50]:
scenarios["Residential with Prioritised Demand"] = setup_and_start_project("Residential with Prioritised Demand", with_residual_storage=True)
[51]:
wait_till_scenario_done(scenarios["Residential with Prioritised Demand"])
2020-05-25 21:57:42.958608 {'status': 6, 'status_text': 'PA_RUNNING'}
2020-05-25 21:57:48.141734 Scenario complete
[52]:
# Download results
scenario_results = get_results(scenarios["Residential with Prioritised Demand"], ['parcel', 'wb_soil', 'wb_lot_storages'])
lot_residential_with_res = pd.DataFrame(scenario_results['parcel'])
lot_residential_with_res = calcuate_mass_balance(lot_residential_with_res)
print_parcel_mass_balance(lot_residential_with_res)
area | persons | impervious_area | roof_area | garden_area | outdoor_imp | potable_demand | outdoor_demand | non_potable_demand | grey_water | black_water | rainfall | evapotranspiration | impervious_runoff | pervious_runoff | roof_runoff | infiltration | mass_balance_runoff_only | mass_balance | wb_lot_template_id | |
---|---|---|---|---|---|---|---|---|---|---|---|---|---|---|---|---|---|---|---|---|
0 | 628.974360 | 4.0 | None | 377.384616 | 150.953846 | 100.635898 | 112.728 | 28.125470 | 6.408272 | 112.728 | 27.816 | 396.002257 | 192.525340 | 46.380798 | 4.129480 | 116.326747 | 42.074310 | 2.269105e+01 | 1.283325e+00 | 1 |
1 | 639.210270 | 2.0 | None | 383.526162 | 153.410465 | 102.273643 | 56.364 | 26.999120 | 2.926000 | 56.364 | 13.908 | 402.446786 | 195.658491 | 47.135598 | 4.196683 | 127.177374 | 42.759026 | 1.251873e+01 | 1.536734e+00 | 1 |
2 | 644.454460 | 2.0 | None | 386.672676 | 154.669070 | 103.112714 | 56.364 | 27.331529 | 2.926000 | 56.364 | 13.908 | 405.748528 | 197.263707 | 47.522306 | 4.231113 | 128.460288 | 43.109828 | 1.249282e+01 | 1.510815e+00 | 1 |
3 | 644.201990 | 1.0 | None | 386.521194 | 154.608478 | 103.072318 | 28.182 | 26.593526 | 1.444000 | 28.182 | 6.954 | 405.589573 | 197.186427 | 47.503689 | 4.229456 | 132.996525 | 43.092939 | 7.174063e+00 | 1.664063e+00 | 1 |
4 | 644.454460 | 4.0 | None | 386.672676 | 154.669070 | 103.112714 | 112.728 | 29.124408 | 6.536000 | 112.728 | 27.816 | 405.748528 | 197.263707 | 47.522306 | 4.231113 | 120.259166 | 43.109828 | 2.248682e+01 | 1.206815e+00 | 1 |
5 | 644.033676 | 2.0 | None | 386.420206 | 154.568082 | 103.045388 | 56.364 | 27.304858 | 2.926000 | 56.364 | 13.908 | 405.483603 | 197.134908 | 47.491277 | 4.228351 | 128.357350 | 43.081680 | 1.249489e+01 | 1.512895e+00 | 1 |
6 | 644.454460 | 4.0 | None | 386.672676 | 154.669070 | 103.112714 | 112.728 | 29.124408 | 6.536000 | 112.728 | 27.816 | 405.748528 | 197.263707 | 47.522306 | 4.231113 | 120.259166 | 43.109828 | 2.248682e+01 | 1.206815e+00 | 1 |
7 | 643.865363 | 2.0 | None | 386.319218 | 154.527687 | 103.018458 | 56.364 | 27.294189 | 2.926000 | 56.364 | 13.908 | 405.377633 | 197.083388 | 47.478866 | 4.227246 | 128.316174 | 43.070421 | 1.249573e+01 | 1.513727e+00 | 1 |
8 | 644.454460 | 3.0 | None | 386.672676 | 154.669070 | 103.112714 | 84.546 | 28.149145 | 4.560000 | 84.546 | 20.862 | 405.748528 | 197.263707 | 47.522306 | 4.231113 | 124.109904 | 43.109828 | 1.766082e+01 | 1.358815e+00 | 1 |
9 | 643.697050 | 1.0 | None | 386.218230 | 154.487292 | 102.991528 | 28.182 | 26.561520 | 1.444000 | 28.182 | 6.954 | 405.271662 | 197.031868 | 47.466454 | 4.226141 | 132.872999 | 43.059162 | 7.176558e+00 | 1.666558e+00 | 1 |
10 | 644.454460 | 2.0 | None | 386.672676 | 154.669070 | 103.112714 | 56.364 | 27.331529 | 2.926000 | 56.364 | 13.908 | 405.748528 | 197.263707 | 47.522306 | 4.231113 | 128.460288 | 43.109828 | 1.249282e+01 | 1.510815e+00 | 1 |
11 | 643.528736 | 3.0 | None | 386.117242 | 154.446897 | 102.964598 | 84.546 | 28.084894 | 4.560000 | 84.546 | 20.862 | 405.165692 | 196.980348 | 47.454043 | 4.225036 | 123.877866 | 43.047903 | 1.766539e+01 | 1.363390e+00 | 1 |
12 | 644.454460 | 3.0 | None | 386.672676 | 154.669070 | 103.112714 | 84.546 | 28.149145 | 4.560000 | 84.546 | 20.862 | 405.748528 | 197.263707 | 47.522306 | 4.231113 | 124.109904 | 43.109828 | 1.766082e+01 | 1.358815e+00 | 1 |
13 | 643.360423 | 1.0 | None | 386.016254 | 154.406502 | 102.937668 | 28.182 | 26.540183 | 1.444000 | 28.182 | 6.954 | 405.059722 | 196.928829 | 47.441631 | 4.223931 | 132.790648 | 43.036644 | 7.178222e+00 | 1.668222e+00 | 1 |
14 | 644.454460 | 3.0 | None | 386.672676 | 154.669070 | 103.112714 | 84.546 | 28.149145 | 4.560000 | 84.546 | 20.862 | 405.748528 | 197.263707 | 47.522306 | 4.231113 | 124.109904 | 43.109828 | 1.766082e+01 | 1.358815e+00 | 1 |
15 | 643.192110 | 4.0 | None | 385.915266 | 154.366106 | 102.910738 | 112.728 | 29.032532 | 6.536000 | 112.728 | 27.816 | 404.953752 | 196.877309 | 47.429220 | 4.222825 | 119.938491 | 43.025385 | 2.249305e+01 | 1.213054e+00 | 1 |
16 | 644.454460 | 2.0 | None | 386.672676 | 154.669070 | 103.112714 | 56.364 | 27.331529 | 2.926000 | 56.364 | 13.908 | 405.748528 | 197.263707 | 47.522306 | 4.231113 | 128.460288 | 43.109828 | 1.249282e+01 | 1.510815e+00 | 1 |
17 | 643.023796 | 2.0 | None | 385.814278 | 154.325711 | 102.883807 | 56.364 | 27.240845 | 2.926000 | 56.364 | 13.908 | 404.847782 | 196.825789 | 47.416808 | 4.221720 | 128.110297 | 43.014126 | 1.249989e+01 | 1.517886e+00 | 1 |
18 | 644.454460 | 1.0 | None | 386.672676 | 154.669070 | 103.112714 | 28.182 | 26.609529 | 1.444000 | 28.182 | 6.954 | 405.748528 | 197.263707 | 47.522306 | 4.231113 | 133.058288 | 43.109828 | 7.172815e+00 | 1.662815e+00 | 1 |
19 | 642.855483 | 3.0 | None | 385.713290 | 154.285316 | 102.856877 | 84.546 | 28.038166 | 4.560000 | 84.546 | 20.862 | 404.741812 | 196.774270 | 47.404397 | 4.220615 | 123.709112 | 43.002867 | 1.766872e+01 | 1.366718e+00 | 1 |
20 | 644.454460 | 1.0 | None | 386.672676 | 154.669070 | 103.112714 | 28.182 | 26.609529 | 1.444000 | 28.182 | 6.954 | 405.748528 | 197.263707 | 47.522306 | 4.231113 | 133.058288 | 43.109828 | 7.172815e+00 | 1.662815e+00 | 1 |
21 | 642.687170 | 2.0 | None | 385.612302 | 154.244921 | 102.829947 | 56.364 | 27.219508 | 2.926000 | 56.364 | 13.908 | 404.635842 | 196.722750 | 47.391985 | 4.219510 | 128.027947 | 42.991608 | 1.250155e+01 | 1.519550e+00 | 1 |
22 | 644.454460 | 2.0 | None | 386.672676 | 154.669070 | 103.112714 | 56.364 | 27.331529 | 2.926000 | 56.364 | 13.908 | 405.748528 | 197.263707 | 47.522306 | 4.231113 | 128.460288 | 43.109828 | 1.249282e+01 | 1.510815e+00 | 1 |
23 | 642.518856 | 2.0 | None | 385.511314 | 154.204526 | 102.803017 | 56.364 | 27.208839 | 2.926000 | 56.364 | 13.908 | 404.529872 | 196.671230 | 47.379574 | 4.218405 | 127.986771 | 42.980349 | 1.250238e+01 | 1.520382e+00 | 1 |
24 | 644.454460 | 4.0 | None | 386.672676 | 154.669070 | 103.112714 | 112.728 | 29.124408 | 6.536000 | 112.728 | 27.816 | 405.748528 | 197.263707 | 47.522306 | 4.231113 | 120.259166 | 43.109828 | 2.248682e+01 | 1.206815e+00 | 1 |
25 | 642.350543 | 4.0 | None | 385.410326 | 154.164130 | 102.776087 | 112.728 | 28.971281 | 6.536000 | 112.728 | 27.816 | 404.423902 | 196.619710 | 47.367162 | 4.217300 | 119.724707 | 42.969090 | 2.249721e+01 | 1.217214e+00 | 1 |
26 | 644.454460 | 3.0 | None | 386.672676 | 154.669070 | 103.112714 | 84.546 | 28.149145 | 4.560000 | 84.546 | 20.862 | 405.748528 | 197.263707 | 47.522306 | 4.231113 | 124.109904 | 43.109828 | 1.766082e+01 | 1.358815e+00 | 1 |
27 | 642.182230 | 3.0 | None | 385.309338 | 154.123735 | 102.749157 | 84.546 | 27.991438 | 4.560000 | 84.546 | 20.862 | 404.317932 | 196.568191 | 47.354751 | 4.216195 | 123.540358 | 42.957831 | 1.767205e+01 | 1.370045e+00 | 1 |
28 | 629.627695 | 2.0 | None | 377.776617 | 151.110647 | 100.740431 | 56.364 | 26.429717 | 2.888000 | 56.364 | 13.908 | 396.413597 | 192.725322 | 46.428975 | 4.133769 | 124.833139 | 42.118013 | 1.260410e+01 | 1.584096e+00 | 1 |
29 | 597.765026 | 4.0 | None | 358.659016 | 143.463606 | 95.642404 | 112.728 | 26.237304 | 6.247946 | 112.728 | 27.816 | 376.352860 | 182.972347 | 44.079410 | 3.924578 | 108.621593 | 39.986607 | 2.300563e+01 | 1.437575e+00 | 1 |
30 | 4920.163308 | NaN | None | NaN | NaN | 2043.436272 | 0.000 | 0.000000 | 0.000000 | 0.000 | 0.000 | 3097.734818 | 1275.455995 | 941.773332 | 78.695489 | 0.000000 | 801.810002 | -1.818989e-12 | -1.818989e-12 | 1 |
Storage behaviour RWHT¶
[53]:
# Plot lot storage 1
lot_storages = pd.DataFrame(scenario_results['wb_lot_storages'])
plot_timeseries_df(lot_storages.loc[0], ['storage_behaviour', 'provided_volume'])
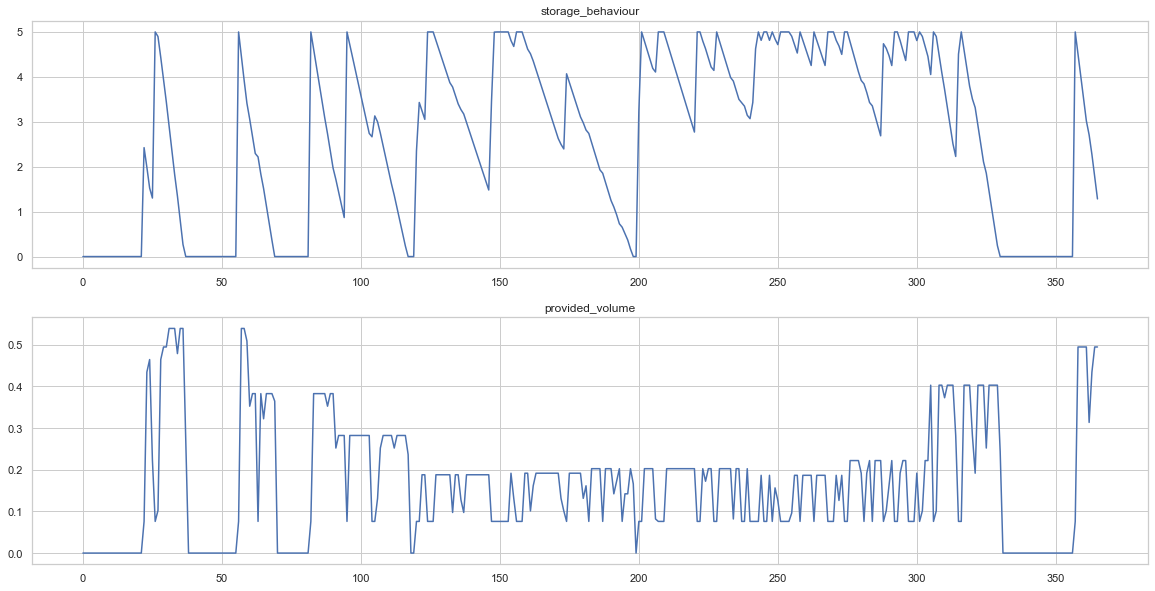
[54]:
# print_parcel_mass_balance(lot_residential_with_res)
Comparison¶
The plot below shows a comparison of most important streams for the above scenarios. Note that the number of persons per residential unit is stochastically generated. Therefore demands can slightly vary accross scenarios.
[55]:
# Append and generate data
ress = [lot_residential, lot_residential_with_rwht, lot_residential_with_grey]
f, axes = plt.subplots(3, 2, figsize=(20, 7));
for r in ress:
inflow(r)
outflow(r)
lot_residential["type"] = "lot_residential"
lot_residential_with_rwht["type"] = "lot_residential_with_rwht"
lot_residential_with_grey["type"] = "lot_residential_with_grey"
lot_residential_with_res["type"] = "lot_residential_with_res"
ress_df = lot_residential.append(lot_residential_with_rwht).append(lot_residential_with_grey).append(lot_residential_with_res)
def plot_results():
display_ress = ["inflow", "outflow", "outdoor_demand", "roof_runoff", "grey_water", "provided_volume"]
for idx, p in enumerate(display_ress):
sns.catplot(x="type", y=p, data=ress_df,
height=6, kind="bar", palette="muted", ax=axes[ int(idx / 2), (idx) % 2]);
for i in range(len(display_ress)):
plt.close(i+2)
plot_results()
/Users/christianurich/Documents/scenario-tool-nodes/.venv/lib/python3.7/site-packages/pandas/core/frame.py:7116: FutureWarning: Sorting because non-concatenation axis is not aligned. A future version
of pandas will change to not sort by default.
To accept the future behavior, pass 'sort=False'.
To retain the current behavior and silence the warning, pass 'sort=True'.
sort=sort,
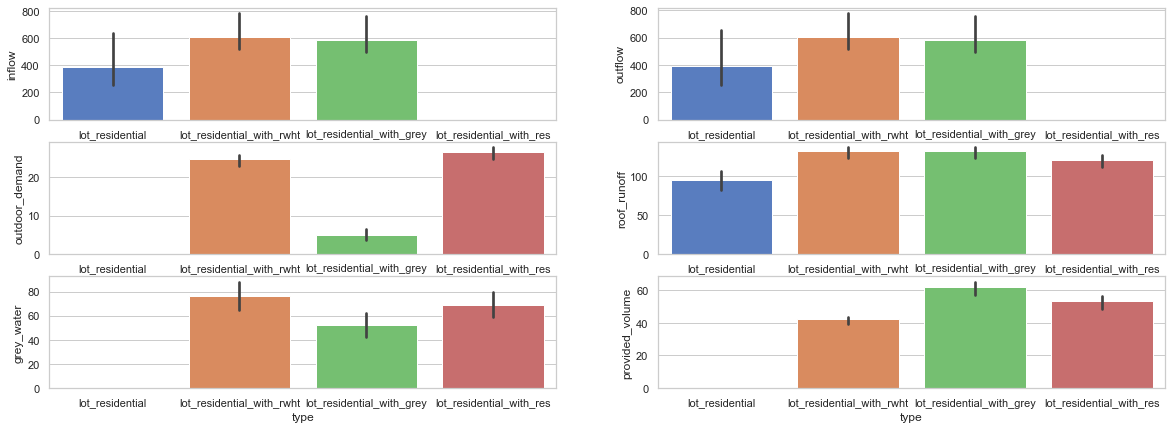
Lot Stream Aggregation¶
Based on the template definition streams are aggregated at lot scale to be connected to sub-catchments. Following streams are used in the water cycle model.
potable_demand
non_potable_demand
outdoor_demand
sewerage
grey_water
stormwater_runoff
evapotranspiration
infiltration
rainfall
Default streams in the model¶
[56]:
# Default stream flow definitions
wb_lot_streams = get_results(baseline_id, ['wb_lot_streams'])
for row in wb_lot_streams['wb_lot_streams']:
print(f"{LotStream(row['lot_stream_id'])} -> {Streams(row['outflow_stream_id'])}")
LotStream.rainfall -> Streams.rainfall
LotStream.potable_demand -> Streams.potable_demand
LotStream.non_potable_demand -> Streams.potable_demand
LotStream.outdoor_demand -> Streams.potable_demand
LotStream.black_water -> Streams.sewerage
LotStream.grey_water -> Streams.sewerage
LotStream.roof_runoff -> Streams.stormwater_runoff
LotStream.impervious_runoff -> Streams.stormwater_runoff
LotStream.evapotranspiration -> Streams.evapotranspiration
LotStream.infiltration -> Streams.infiltration
Sub Catchments¶
This scenario sets up a small residential development next to a public green space. It simulates how water can be exported form the residential development to reduce the outdoor demand.
[57]:
def catchment_streams(catchment_id, stream_id):
catchments = {
"node_type_id": sti.get_node_id("Sub Catchment"),
"area": geojson_id_right,
"parameters":
{
"dance4water_stream.equation" : stream_id,
"dance4water_catchment_id.equation": catchment_id
}
}
return catchments
[58]:
# Setup Subcatchment without storage
with open(r"../../resources/boundaries/test_small_left.geojson", 'r') as file:
geojson_file = json.loads(file.read())
geojson_id_left = sti.upload_geojson(geojson_file, project_id, "left")
with open(r"../../resources/boundaries/test_small_right.geojson", 'r') as file:
geojson_file = json.loads(file.read())
geojson_id_right = sti.upload_geojson(geojson_file, project_id, "right")
nodes = [{
"node_type_id": sti.get_node_id("Residential"),
"area": geojson_id_left,
"parameters":
{
}
}, {
"node_type_id": sti.get_node_id("Clear area"),
"area": geojson_id_right,
"parameters":
{
}
}, {
"node_type_id": sti.get_node_id("Copy Feature"),
"area": geojson_id_right,
"parameters":
{
"dance4water_copy_feature.to_view": "parcel_tmp"
}
}, {
"node_type_id": sti.get_node_id("SQL query"),
"area": geojson_id_right,
"parameters":
{
"dance4water_sql_query.attribute": "parcel_tmp.area",
"dance4water_sql_query.query": f"UPDATE parcel_tmp SET area = ST_AREA(GEOMETRY)",
"dance4water_sql_query.attribute_type": "DOUBLE"
}
},
{
"node_type_id": sti.get_node_id("SQL query"),
"area": geojson_id_right,
"parameters":
{
"dance4water_sql_query.attribute": "parcel_tmp.garden_area",
"dance4water_sql_query.query": f"UPDATE parcel_tmp SET garden_area = ST_AREA(GEOMETRY)",
"dance4water_sql_query.attribute_type": "DOUBLE"
}
}, {
"node_type_id": sti.get_node_id("Copy Feature"),
"area": geojson_id_right,
"parameters":
{
"dance4water_copy_feature.from_view": "parcel_tmp",
"dance4water_copy_feature.to_view": "parcel"
}
},{
"node_type_id": sti.get_node_id("Lot Template"),
"area": geojson_id_right,
"parameters":
{
"dance4water_outdoor_demand.equation": 3
}
}
]
nodes.append(catchment_streams(2,3))
# nodes += wb_catchment("default", geojson_id_left)
nodes += wb_catchment("Outdoor area", geojson_id_right)
scenario_1 = sti.create_scenario(project_id, baseline_id, "Split Catchment")
sti.set_scenario_workflow(scenario_1, nodes)
sti.execute_scenario(scenario_1)
[59]:
wait_till_scenario_done(scenario_1)
2020-05-25 21:59:08.712641 {'status': 6, 'status_text': 'PA_RUNNING'}
2020-05-25 21:59:14.270974 Scenario complete
[60]:
scenario_results = get_results(scenario_1, ['parcel', 'wb_soil', 'wb_lot_storages', 'wb_sub_catchment', 'wb_storages', 'wb_sub_storages'])
scenario_1_pd = pd.DataFrame(scenario_results['parcel'])
scenario_1_pd = calcuate_mass_balance(scenario_1_pd)
print_parcel_mass_balance(scenario_1_pd)
area | persons | impervious_area | roof_area | garden_area | outdoor_imp | potable_demand | outdoor_demand | non_potable_demand | grey_water | black_water | rainfall | evapotranspiration | impervious_runoff | pervious_runoff | roof_runoff | infiltration | mass_balance_runoff_only | mass_balance | wb_lot_template_id | |
---|---|---|---|---|---|---|---|---|---|---|---|---|---|---|---|---|---|---|---|---|
0 | 474.421724 | 2.0 | None | 284.653034 | 113.861214 | 75.907476 | 56.364 | 51.157548 | 13.908 | 56.364 | 13.908 | 298.695917 | 145.217690 | 34.984030 | 3.114777 | 134.801228 | 31.735740 | 4.014566e-13 | 4.014566e-13 | 1 |
1 | 582.576208 | 4.0 | None | 349.545725 | 139.818290 | 93.212193 | 112.728 | 62.819995 | 27.816 | 112.728 | 27.816 | 366.789980 | 178.323139 | 42.959381 | 3.824857 | 165.532024 | 38.970574 | 9.237056e-14 | 3.552714e-14 | 1 |
2 | 636.777013 | 3.0 | None | 382.066208 | 152.826483 | 101.884322 | 84.546 | 68.664542 | 20.862 | 84.546 | 20.862 | 400.914807 | 194.913686 | 46.956168 | 4.180708 | 180.932531 | 42.596257 | -5.044853e-13 | -4.476419e-13 | 1 |
3 | 636.529886 | 1.0 | None | 381.917932 | 152.767173 | 101.844782 | 28.182 | 68.637894 | 6.954 | 28.182 | 6.954 | 400.759216 | 194.838042 | 46.937945 | 4.179085 | 180.862313 | 42.579725 | -2.700062e-13 | -2.700062e-13 | 1 |
4 | 636.777013 | 2.0 | None | 382.066208 | 152.826483 | 101.884322 | 56.364 | 68.664542 | 13.908 | 56.364 | 13.908 | 400.914807 | 194.913686 | 46.956168 | 4.180708 | 180.932531 | 42.596257 | -8.739676e-13 | -8.739676e-13 | 1 |
5 | 636.365135 | 1.0 | None | 381.819081 | 152.727632 | 101.818422 | 28.182 | 68.620129 | 6.954 | 28.182 | 6.954 | 400.655489 | 194.787612 | 46.925796 | 4.178004 | 180.815501 | 42.568705 | 2.273737e-13 | 2.273737e-13 | 1 |
6 | 636.777013 | 4.0 | None | 382.066208 | 152.826483 | 101.884322 | 112.728 | 68.664542 | 27.816 | 112.728 | 27.816 | 400.914807 | 194.913686 | 46.956168 | 4.180708 | 180.932531 | 42.596257 | -7.105427e-14 | -7.105427e-14 | 1 |
7 | 636.200384 | 3.0 | None | 381.720231 | 152.688092 | 101.792061 | 84.546 | 68.602364 | 20.862 | 84.546 | 20.862 | 400.551762 | 194.737183 | 46.913648 | 4.176922 | 180.768689 | 42.557684 | 4.121148e-13 | 4.121148e-13 | 1 |
8 | 636.777013 | 2.0 | None | 382.066208 | 152.826483 | 101.884322 | 56.364 | 68.664542 | 13.908 | 56.364 | 13.908 | 400.914807 | 194.913686 | 46.956168 | 4.180708 | 180.932531 | 42.596257 | 3.907985e-13 | 3.907985e-13 | 1 |
9 | 636.035633 | 3.0 | None | 381.621380 | 152.648552 | 101.765701 | 84.546 | 68.584598 | 20.862 | 84.546 | 20.862 | 400.448035 | 194.686754 | 46.901499 | 4.175840 | 180.721877 | 42.546663 | -8.668621e-13 | -8.668621e-13 | 1 |
10 | 636.777013 | 1.0 | None | 382.066208 | 152.826483 | 101.884322 | 28.182 | 68.664542 | 6.954 | 28.182 | 6.954 | 400.914807 | 194.913686 | 46.956168 | 4.180708 | 180.932531 | 42.596257 | -3.765876e-13 | -3.765876e-13 | 1 |
11 | 635.870882 | 4.0 | None | 381.522529 | 152.609012 | 101.739341 | 112.728 | 68.566833 | 27.816 | 112.728 | 27.816 | 400.344308 | 194.636325 | 46.889350 | 4.174759 | 180.675065 | 42.535642 | -2.131628e-13 | -2.131628e-13 | 1 |
12 | 621.988433 | 4.0 | None | 373.193060 | 149.277224 | 99.518149 | 112.728 | 67.069869 | 27.816 | 112.728 | 27.816 | 391.603917 | 190.386989 | 45.865653 | 4.083614 | 176.730534 | 41.606996 | -6.323830e-13 | -6.892265e-13 | 1 |
13 | 591.446768 | 4.0 | None | 354.868061 | 141.947224 | 94.631483 | 112.728 | 63.776519 | 27.816 | 112.728 | 27.816 | 372.374885 | 181.038365 | 43.613500 | 3.883096 | 168.052487 | 39.563957 | 0.000000e+00 | -5.684342e-14 | 1 |
14 | 12491.101688 | NaN | None | NaN | 12491.101688 | NaN | 0.000 | 5612.219568 | 0.000 | 0.000 | 0.000 | 7864.397623 | 9653.354334 | 0.000000 | 341.705465 | 0.000000 | 3481.557391 | 1.409717e-11 | 1.409717e-11 | 2 |
15 | 3005.815971 | NaN | None | NaN | NaN | 9742.245511 | 0.000 | 0.000000 | 0.000 | 0.000 | 0.000 | 1892.461735 | -535.638743 | 4489.979525 | -184.281167 | 0.000000 | -1877.597881 | 4.547474e-13 | 4.547474e-13 | 1 |
16 | NaN | NaN | None | NaN | NaN | NaN | 0.000 | 0.000000 | 0.000 | 0.000 | 0.000 | 0.000000 | 0.000000 | 0.000000 | 0.000000 | 0.000000 | 0.000000 | 0.000000e+00 | 0.000000e+00 | 1 |
[61]:
pd.DataFrame(scenario_results['wb_sub_catchment'])
[61]:
annual_flow | catchment_id | daily_flow | ogc_fid | stream | |
---|---|---|---|---|---|
0 | 4532.652920 | 1 | [17.1369, 18.7949, 18.7949, 7.296, 12.992, 18.... | 1 | 1 |
1 | 1335.168000 | 1 | [3.648, 3.648, 3.648, 3.648, 3.648, 3.648, 3.6... | 2 | 4 |
2 | 7922.079010 | 1 | [0.0, 0.0, 0.0, 0.0, 0.0, 0.0, 0.0, 0.0, 0.0, ... | 3 | 6 |
3 | 11760.936118 | 1 | [44.2296, 40.4022, 40.4022, 82.0496, 53.7983, ... | 4 | 7 |
4 | 2181.606479 | 1 | [0.0, 0.0, 0.0, 0.0, 0.0, 0.0, 0.0, 0.0, 0.0, ... | 5 | 8 |
5 | 15193.656904 | 1 | [9.6529, 0.0, 0.0, 82.0496, 33.7851, 0.0, 0.0,... | 6 | 9 |
6 | 0.000000 | 1 | [0.0, 0.0, 0.0, 0.0, 0.0, 0.0, 0.0, 0.0, 0.0, ... | 7 | 2 |
7 | 0.000000 | 1 | [0.0, 0.0, 0.0, 0.0, 0.0, 0.0, 0.0, 0.0, 0.0, ... | 8 | 3 |
8 | 0.000000 | 1 | [0.0, 0.0, 0.0, 0.0, 0.0, 0.0, 0.0, 0.0, 0.0, ... | 9 | 5 |
9 | 5612.219568 | 2 | [29.6563, 34.6527, 34.6527, 0.0, 17.1652, 34.6... | 10 | 3 |
[62]:
scenario_results['wb_sub_storages']
[62]:
[]
[63]:
# Setup Subcatchment with storage
with open(r"../../resources/boundaries/test_small_left.geojson", 'r') as file:
geojson_file = json.loads(file.read())
geojson_id_left = sti.upload_geojson(geojson_file, project_id, "left")
with open(r"../../resources/boundaries/test_small_right.geojson", 'r') as file:
geojson_file = json.loads(file.read())
geojson_id_right = sti.upload_geojson(geojson_file, project_id, "right")
nodes = [{
"node_type_id": sti.get_node_id("Residential"),
"area": geojson_id_left,
"parameters":
{
}
}, {
"node_type_id": sti.get_node_id("Clear area"),
"area": geojson_id_right,
"parameters":
{
}
}, {
"node_type_id": sti.get_node_id("Copy Feature"),
"area": geojson_id_right,
"parameters":
{
"dance4water_copy_feature.to_view": "parcel_tmp"
}
}, {
"node_type_id": sti.get_node_id("SQL query"),
"area": geojson_id_right,
"parameters":
{
"dance4water_sql_query.attribute": "parcel_tmp.area",
"dance4water_sql_query.query": f"UPDATE parcel_tmp SET area = ST_AREA(GEOMETRY)",
"dance4water_sql_query.attribute_type": "DOUBLE"
}
},
{
"node_type_id": sti.get_node_id("SQL query"),
"area": geojson_id_right,
"parameters":
{
"dance4water_sql_query.attribute": "parcel_tmp.garden_area",
"dance4water_sql_query.query": f"UPDATE parcel_tmp SET garden_area = ST_AREA(GEOMETRY)",
"dance4water_sql_query.attribute_type": "DOUBLE"
}
}, {
"node_type_id": sti.get_node_id("Copy Feature"),
"area": geojson_id_right,
"parameters":
{
"dance4water_copy_feature.from_view": "parcel_tmp",
"dance4water_copy_feature.to_view": "parcel"
}
},{
"node_type_id": sti.get_node_id("Lot Template"),
"area": geojson_id_right,
"parameters":
{
"dance4water_outdoor_demand.equation": 3
}
},{
"node_type_id": sti.get_node_id("Sub Catchment Storage"),
"area": geojson_id_right,
"parameters":
{
"dance4water_inflow_stream.equation": 3,
"dance4water_demand_stream.equation": 7,
"dance4water_storage_volume.equation": 50,
}
}
]
nodes.append(catchment_streams(2,3))
scenario_2 = sti.create_scenario(project_id, baseline_id, "test sub")
# nodes += wb_catchment("default", geojson_id_left)
nodes += wb_catchment("Outdoor area", geojson_id_right)
sti.set_scenario_workflow(scenario_2, nodes)
sti.execute_scenario(scenario_2)
[ ]:
[64]:
wait_till_scenario_done(scenario_2)
2020-05-25 22:00:53.919002 {'status': 6, 'status_text': 'PA_RUNNING'}
2020-05-25 22:00:59.100340 Scenario complete
[65]:
scenario_results_2 = get_results(scenario_2, ['parcel', 'wb_soil', 'wb_lot_storages', 'wb_sub_catchment', 'wb_storages', 'wb_sub_storages'])
scenario_2_pd = pd.DataFrame(scenario_results['parcel'])
scenario_2_pd = calcuate_mass_balance(scenario_2_pd)
print_parcel_mass_balance(scenario_2_pd)
area | persons | impervious_area | roof_area | garden_area | outdoor_imp | potable_demand | outdoor_demand | non_potable_demand | grey_water | black_water | rainfall | evapotranspiration | impervious_runoff | pervious_runoff | roof_runoff | infiltration | mass_balance_runoff_only | mass_balance | wb_lot_template_id | |
---|---|---|---|---|---|---|---|---|---|---|---|---|---|---|---|---|---|---|---|---|
0 | 474.421724 | 2.0 | None | 284.653034 | 113.861214 | 75.907476 | 56.364 | 51.157548 | 13.908 | 56.364 | 13.908 | 298.695917 | 145.217690 | 34.984030 | 3.114777 | 134.801228 | 31.735740 | 4.014566e-13 | 4.014566e-13 | 1 |
1 | 582.576208 | 4.0 | None | 349.545725 | 139.818290 | 93.212193 | 112.728 | 62.819995 | 27.816 | 112.728 | 27.816 | 366.789980 | 178.323139 | 42.959381 | 3.824857 | 165.532024 | 38.970574 | 9.237056e-14 | 3.552714e-14 | 1 |
2 | 636.777013 | 3.0 | None | 382.066208 | 152.826483 | 101.884322 | 84.546 | 68.664542 | 20.862 | 84.546 | 20.862 | 400.914807 | 194.913686 | 46.956168 | 4.180708 | 180.932531 | 42.596257 | -5.044853e-13 | -4.476419e-13 | 1 |
3 | 636.529886 | 1.0 | None | 381.917932 | 152.767173 | 101.844782 | 28.182 | 68.637894 | 6.954 | 28.182 | 6.954 | 400.759216 | 194.838042 | 46.937945 | 4.179085 | 180.862313 | 42.579725 | -2.700062e-13 | -2.700062e-13 | 1 |
4 | 636.777013 | 2.0 | None | 382.066208 | 152.826483 | 101.884322 | 56.364 | 68.664542 | 13.908 | 56.364 | 13.908 | 400.914807 | 194.913686 | 46.956168 | 4.180708 | 180.932531 | 42.596257 | -8.739676e-13 | -8.739676e-13 | 1 |
5 | 636.365135 | 1.0 | None | 381.819081 | 152.727632 | 101.818422 | 28.182 | 68.620129 | 6.954 | 28.182 | 6.954 | 400.655489 | 194.787612 | 46.925796 | 4.178004 | 180.815501 | 42.568705 | 2.273737e-13 | 2.273737e-13 | 1 |
6 | 636.777013 | 4.0 | None | 382.066208 | 152.826483 | 101.884322 | 112.728 | 68.664542 | 27.816 | 112.728 | 27.816 | 400.914807 | 194.913686 | 46.956168 | 4.180708 | 180.932531 | 42.596257 | -7.105427e-14 | -7.105427e-14 | 1 |
7 | 636.200384 | 3.0 | None | 381.720231 | 152.688092 | 101.792061 | 84.546 | 68.602364 | 20.862 | 84.546 | 20.862 | 400.551762 | 194.737183 | 46.913648 | 4.176922 | 180.768689 | 42.557684 | 4.121148e-13 | 4.121148e-13 | 1 |
8 | 636.777013 | 2.0 | None | 382.066208 | 152.826483 | 101.884322 | 56.364 | 68.664542 | 13.908 | 56.364 | 13.908 | 400.914807 | 194.913686 | 46.956168 | 4.180708 | 180.932531 | 42.596257 | 3.907985e-13 | 3.907985e-13 | 1 |
9 | 636.035633 | 3.0 | None | 381.621380 | 152.648552 | 101.765701 | 84.546 | 68.584598 | 20.862 | 84.546 | 20.862 | 400.448035 | 194.686754 | 46.901499 | 4.175840 | 180.721877 | 42.546663 | -8.668621e-13 | -8.668621e-13 | 1 |
10 | 636.777013 | 1.0 | None | 382.066208 | 152.826483 | 101.884322 | 28.182 | 68.664542 | 6.954 | 28.182 | 6.954 | 400.914807 | 194.913686 | 46.956168 | 4.180708 | 180.932531 | 42.596257 | -3.765876e-13 | -3.765876e-13 | 1 |
11 | 635.870882 | 4.0 | None | 381.522529 | 152.609012 | 101.739341 | 112.728 | 68.566833 | 27.816 | 112.728 | 27.816 | 400.344308 | 194.636325 | 46.889350 | 4.174759 | 180.675065 | 42.535642 | -2.131628e-13 | -2.131628e-13 | 1 |
12 | 621.988433 | 4.0 | None | 373.193060 | 149.277224 | 99.518149 | 112.728 | 67.069869 | 27.816 | 112.728 | 27.816 | 391.603917 | 190.386989 | 45.865653 | 4.083614 | 176.730534 | 41.606996 | -6.323830e-13 | -6.892265e-13 | 1 |
13 | 591.446768 | 4.0 | None | 354.868061 | 141.947224 | 94.631483 | 112.728 | 63.776519 | 27.816 | 112.728 | 27.816 | 372.374885 | 181.038365 | 43.613500 | 3.883096 | 168.052487 | 39.563957 | 0.000000e+00 | -5.684342e-14 | 1 |
14 | 12491.101688 | NaN | None | NaN | 12491.101688 | NaN | 0.000 | 5612.219568 | 0.000 | 0.000 | 0.000 | 7864.397623 | 9653.354334 | 0.000000 | 341.705465 | 0.000000 | 3481.557391 | 1.409717e-11 | 1.409717e-11 | 2 |
15 | 3005.815971 | NaN | None | NaN | NaN | 9742.245511 | 0.000 | 0.000000 | 0.000 | 0.000 | 0.000 | 1892.461735 | -535.638743 | 4489.979525 | -184.281167 | 0.000000 | -1877.597881 | 4.547474e-13 | 4.547474e-13 | 1 |
16 | NaN | NaN | None | NaN | NaN | NaN | 0.000 | 0.000000 | 0.000 | 0.000 | 0.000 | 0.000000 | 0.000000 | 0.000000 | 0.000000 | 0.000000 | 0.000000 | 0.000000e+00 | 0.000000e+00 | 1 |
[66]:
pd.DataFrame(scenario_results_2['wb_sub_catchment'])
[66]:
annual_flow | catchment_id | daily_flow | ogc_fid | stream | |
---|---|---|---|---|---|
0 | 4251.564920 | 1 | [16.3689, 18.0269, 18.0269, 6.528, 12.224, 18.... | 1 | 1 |
1 | 1194.624000 | 1 | [3.264, 3.264, 3.264, 3.264, 3.264, 3.264, 3.2... | 2 | 4 |
2 | 7872.079010 | 1 | [0.0, 0.0, 0.0, 0.0, 0.0, 0.0, 0.0, 0.0, 0.0, ... | 3 | 6 |
3 | 11760.936118 | 1 | [44.2296, 40.4022, 40.4022, 82.0496, 53.7983, ... | 4 | 7 |
4 | 2181.606479 | 1 | [0.0, 0.0, 0.0, 0.0, 0.0, 0.0, 0.0, 0.0, 0.0, ... | 5 | 8 |
5 | 15193.656904 | 1 | [9.6529, 0.0, 0.0, 82.0496, 33.7851, 0.0, 0.0,... | 6 | 9 |
6 | 0.000000 | 1 | [0.0, 0.0, 0.0, 0.0, 0.0, 0.0, 0.0, 0.0, 0.0, ... | 7 | 2 |
7 | 0.000000 | 1 | [0.0, 0.0, 0.0, 0.0, 0.0, 0.0, 0.0, 0.0, 0.0, ... | 8 | 3 |
8 | 0.000000 | 1 | [0.0, 0.0, 0.0, 0.0, 0.0, 0.0, 0.0, 0.0, 0.0, ... | 9 | 5 |
9 | 5612.219568 | 2 | [29.6563, 34.6527, 34.6527, 0.0, 17.1652, 34.6... | 10 | 3 |
[67]:
scenario_results_2['wb_sub_storages']
[67]:
[{'demand_stream_id': 7,
'dry': 22,
'inflow_stream_id': 3,
'ogc_fid': 1,
'provided_volume': array([0., 0., 0., 0., 0., 0., 0., 0., 0., 0., 0., 0., 0., 0., 0., 0., 0.,
0., 0., 0., 0., 0., 0., 0., 0., 0., 0., 0., 0., 0., 0., 0., 0., 0.,
0., 0., 0., 0., 0., 0., 0., 0., 0., 0., 0., 0., 0., 0., 0., 0., 0.,
0., 0., 0., 0., 0., 0., 0., 0., 0., 0., 0., 0., 0., 0., 0., 0., 0.,
0., 0., 0., 0., 0., 0., 0., 0., 0., 0., 0., 0., 0., 0., 0., 0., 0.,
0., 0., 0., 0., 0., 0., 0., 0., 0., 0., 0., 0., 0., 0., 0., 0., 0.,
0., 0., 0., 0., 0., 0., 0., 0., 0., 0., 0., 0., 0., 0., 0., 0., 0.,
0., 0., 0., 0., 0., 0., 0., 0., 0., 0., 0., 0., 0., 0., 0., 0., 0.,
0., 0., 0., 0., 0., 0., 0., 0., 0., 0., 0., 0., 0., 0., 0., 0., 0.,
0., 0., 0., 0., 0., 0., 0., 0., 0., 0., 0., 0., 0., 0., 0., 0., 0.,
0., 0., 0., 0., 0., 0., 0., 0., 0., 0., 0., 0., 0., 0., 0., 0., 0.,
0., 0., 0., 0., 0., 0., 0., 0., 0., 0., 0., 0., 0., 0., 0., 0., 0.,
0., 0., 0., 0., 0., 0., 0., 0., 0., 0., 0., 0., 0., 0., 0., 0., 0.,
0., 0., 0., 0., 0., 0., 0., 0., 0., 0., 0., 0., 0., 0., 0., 0., 0.,
0., 0., 0., 0., 0., 0., 0., 0., 0., 0., 0., 0., 0., 0., 0., 0., 0.,
0., 0., 0., 0., 0., 0., 0., 0., 0., 0., 0., 0., 0., 0., 0., 0., 0.,
0., 0., 0., 0., 0., 0., 0., 0., 0., 0., 0., 0., 0., 0., 0., 0., 0.,
0., 0., 0., 0., 0., 0., 0., 0., 0., 0., 0., 0., 0., 0., 0., 0., 0.,
0., 0., 0., 0., 0., 0., 0., 0., 0., 0., 0., 0., 0., 0., 0., 0., 0.,
0., 0., 0., 0., 0., 0., 0., 0., 0., 0., 0., 0., 0., 0., 0., 0., 0.,
0., 0., 0., 0., 0., 0., 0., 0., 0., 0., 0., 0., 0., 0., 0., 0., 0.,
0., 0., 0., 0., 0., 0., 0., 0., 0.]),
'spills': 58,
'storage_behaviour': array([ 0., 0., 0., 0., 0., 0., 0., 0., 0., 0., 0., 0., 0.,
0., 0., 0., 0., 0., 0., 0., 0., 0., 50., 50., 50., 50.,
50., 50., 50., 50., 50., 50., 50., 50., 50., 50., 50., 50., 50.,
50., 50., 50., 50., 50., 50., 50., 50., 50., 50., 50., 50., 50.,
50., 50., 50., 50., 50., 50., 50., 50., 50., 50., 50., 50., 50.,
50., 50., 50., 50., 50., 50., 50., 50., 50., 50., 50., 50., 50.,
50., 50., 50., 50., 50., 50., 50., 50., 50., 50., 50., 50., 50.,
50., 50., 50., 50., 50., 50., 50., 50., 50., 50., 50., 50., 50.,
50., 50., 50., 50., 50., 50., 50., 50., 50., 50., 50., 50., 50.,
50., 50., 50., 50., 50., 50., 50., 50., 50., 50., 50., 50., 50.,
50., 50., 50., 50., 50., 50., 50., 50., 50., 50., 50., 50., 50.,
50., 50., 50., 50., 50., 50., 50., 50., 50., 50., 50., 50., 50.,
50., 50., 50., 50., 50., 50., 50., 50., 50., 50., 50., 50., 50.,
50., 50., 50., 50., 50., 50., 50., 50., 50., 50., 50., 50., 50.,
50., 50., 50., 50., 50., 50., 50., 50., 50., 50., 50., 50., 50.,
50., 50., 50., 50., 50., 50., 50., 50., 50., 50., 50., 50., 50.,
50., 50., 50., 50., 50., 50., 50., 50., 50., 50., 50., 50., 50.,
50., 50., 50., 50., 50., 50., 50., 50., 50., 50., 50., 50., 50.,
50., 50., 50., 50., 50., 50., 50., 50., 50., 50., 50., 50., 50.,
50., 50., 50., 50., 50., 50., 50., 50., 50., 50., 50., 50., 50.,
50., 50., 50., 50., 50., 50., 50., 50., 50., 50., 50., 50., 50.,
50., 50., 50., 50., 50., 50., 50., 50., 50., 50., 50., 50., 50.,
50., 50., 50., 50., 50., 50., 50., 50., 50., 50., 50., 50., 50.,
50., 50., 50., 50., 50., 50., 50., 50., 50., 50., 50., 50., 50.,
50., 50., 50., 50., 50., 50., 50., 50., 50., 50., 50., 50., 50.,
50., 50., 50., 50., 50., 50., 50., 50., 50., 50., 50., 50., 50.,
50., 50., 50., 50., 50., 50., 50., 50., 50., 50., 50., 50., 50.,
50., 50., 50., 50., 50., 50., 50., 50., 50., 50., 50., 50., 50.,
50., 50.]),
'volume': 50}]